Hefeng Wu
Paper download is intended for registered attendees only, and is
subjected to the IEEE Copyright Policy. Any other use is strongly forbidden.
Papers from this author
Exploiting Knowledge Embedded Soft Labels for Image Recognition
Lixian Yuan, Riquan Chen, Hefeng Wu, Tianshui Chen, Wentao Wang, Pei Chen
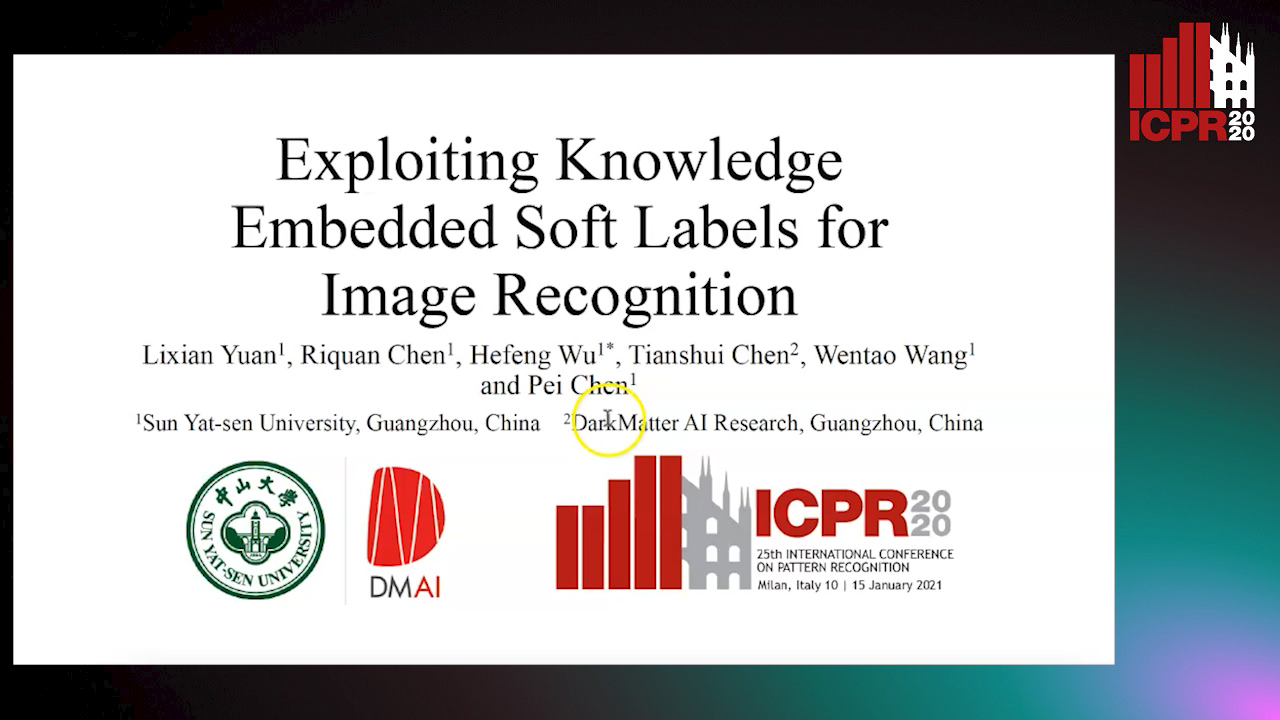
Auto-TLDR; A Soft Label Vector for Image Recognition
Abstract Slides Poster Similar
Objects from correlated classes usually share highly similar appearances while objects from uncorrelated classes are very different. Most of current image recognition works treat each class independently, which ignores these class correlations and inevitably leads to sub-optimal performance in many cases. Fortunately, object classes inherently form a hierarchy with different levels of abstraction and this hierarchy encodes rich correlations among different classes. In this work, we utilize a soft label vector that encodes the prior knowledge of class correlations as extra regularization to train the image classifiers. Specifically, for each class, instead of simply using a one-hot vector, we assign a high value to its correlated classes and assign small values to those uncorrelated ones, thus generating knowledge embedded soft labels. We conduct experiments on both general and fine-grained image recognition benchmarks and demonstrate its superiority compared with existing methods.