Chih-Hsing Ho
Paper download is intended for registered attendees only, and is
subjected to the IEEE Copyright Policy. Any other use is strongly forbidden.
Papers from this author
RSAC: Regularized Subspace Approximation Classifier for Lightweight Continuous Learning
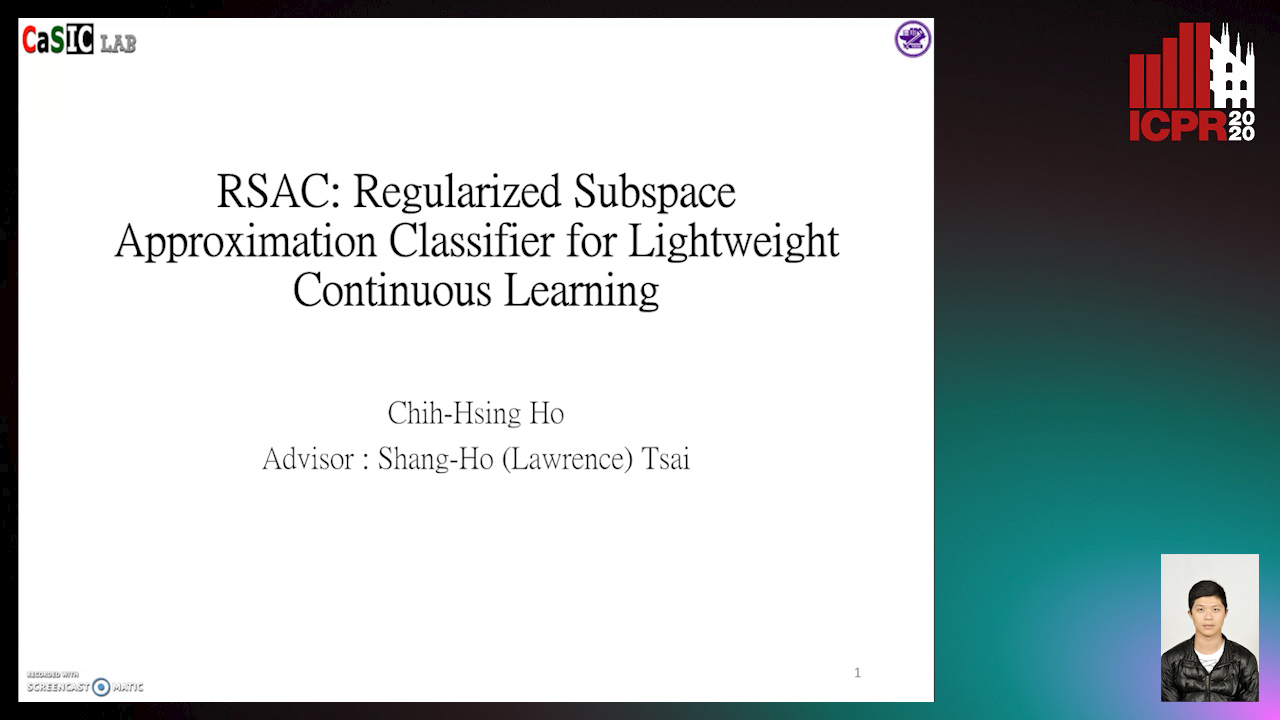
Auto-TLDR; Regularized Subspace Approximation Classifier for Lightweight Continuous Learning
Abstract Slides Poster Similar
Continuous learning seeks to perform the learning on the data that arrives from time to time. While prior works have demonstrated several possible solutions, these approaches require excessive training time as well as memory usage. This is impractical for applications where time and storage are constrained, such as edge computing. In this work, a novel training algorithm, regularized subspace approximation classifier (RSAC), is proposed to achieve lightweight continuous learning. RSAC contains a feature reduction module and classifier module with regularization. Extensive experiments show that RSAC is more efficient than prior continuous learning works and outperforms these works on various experimental settings.