Johannes Haug
Paper download is intended for registered attendees only, and is
subjected to the IEEE Copyright Policy. Any other use is strongly forbidden.
Papers from this author
Learning Parameter Distributions to Detect Concept Drift in Data Streams
Johannes Haug, Gjergji Kasneci
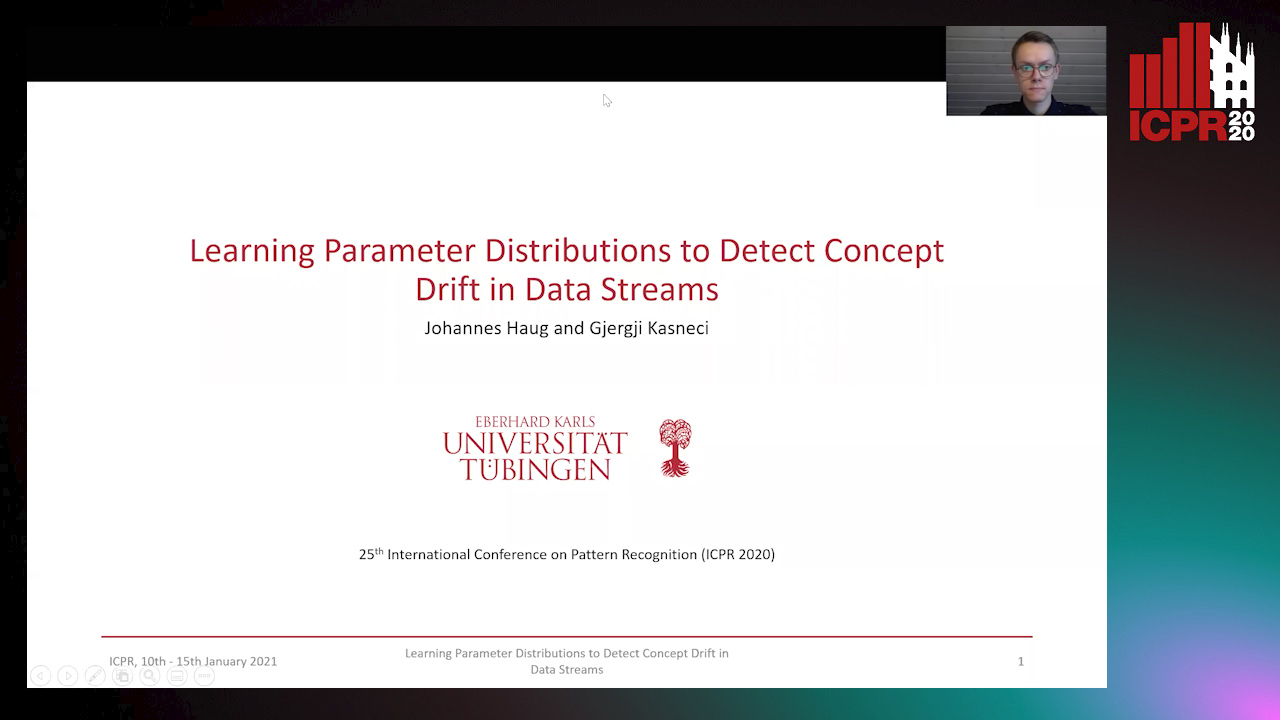
Auto-TLDR; A novel framework for the detection of concept drift in streaming environments
Abstract Slides Poster Similar
Data distributions in streaming environments are usually not stationary. In order to maintain a high predictive quality at all times, online learning models need to adapt to distributional changes, which are known as concept drift. The timely and robust identification of concept drift can be difficult, as we never have access to the true distribution of streaming data. In this work, we propose a novel framework for the detection of real concept drift, called ERICS. By treating the parameters of a predictive model as random variables, we show that concept drift corresponds to a change in the distribution of optimal parameters. To this end, we adopt common measures from information theory. The proposed framework is completely model-agnostic. By choosing an appropriate base model, ERICS is also capable to detect concept drift at the input level, which is a significant advantage over existing approaches. An evaluation on several synthetic and real-world data sets suggests that the proposed framework identifies concept drift more effectively and precisely than various existing works.