Alex Mourer
Paper download is intended for registered attendees only, and is
subjected to the IEEE Copyright Policy. Any other use is strongly forbidden.
Papers from this author
An Invariance-Guided Stability Criterion for Time Series Clustering Validation
Florent Forest, Alex Mourer, Mustapha Lebbah, Hanane Azzag, Jérôme Lacaille
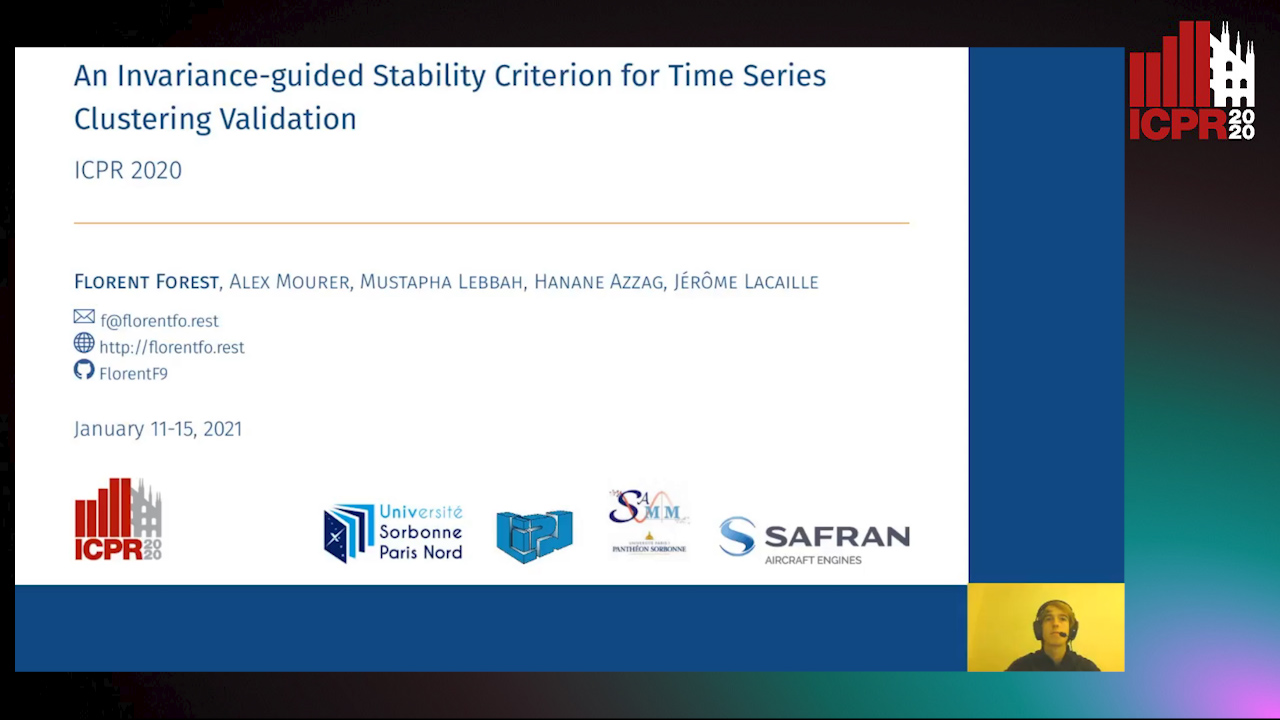
Auto-TLDR; An invariance-guided method for clustering model selection in time series data
Abstract Slides Poster Similar
Time series clustering is a challenging task due to the specificities of this type of data. Temporal correlation and invariance to transformations such as shifting, warping or noise prevent the use of standard data mining methods. Time series clustering has been mostly studied under the angle of finding efficient algorithms and distance metrics adapted to the specific nature of time series data. Much less attention has been devoted to the general problem of model selection. Clustering stability has emerged as a universal and model-agnostic principle for clustering model selection. This principle can be stated as follows: an algorithm should find a structure in the data that is resilient to perturbation by sampling or noise. We propose to apply stability analysis to time series by leveraging prior knowledge on the nature and invariances of the data. These invariances determine the perturbation process used to assess stability. Based on a recently introduced criterion combining between-cluster and within-cluster stability, we propose an invariance-guided method for model selection, applicable to a wide range of clustering algorithms. Experiments conducted on artificial and benchmark data sets demonstrate the ability of our criterion to discover structure and select the correct number of clusters, whenever data invariances are known beforehand.