Stavroula Mougiakakou
Paper download is intended for registered attendees only, and is
subjected to the IEEE Copyright Policy. Any other use is strongly forbidden.
Papers from this author
Partially Supervised Multi-Task Network for Single-View Dietary Assessment
Ya Lu, Thomai Stathopoulou, Stavroula Mougiakakou
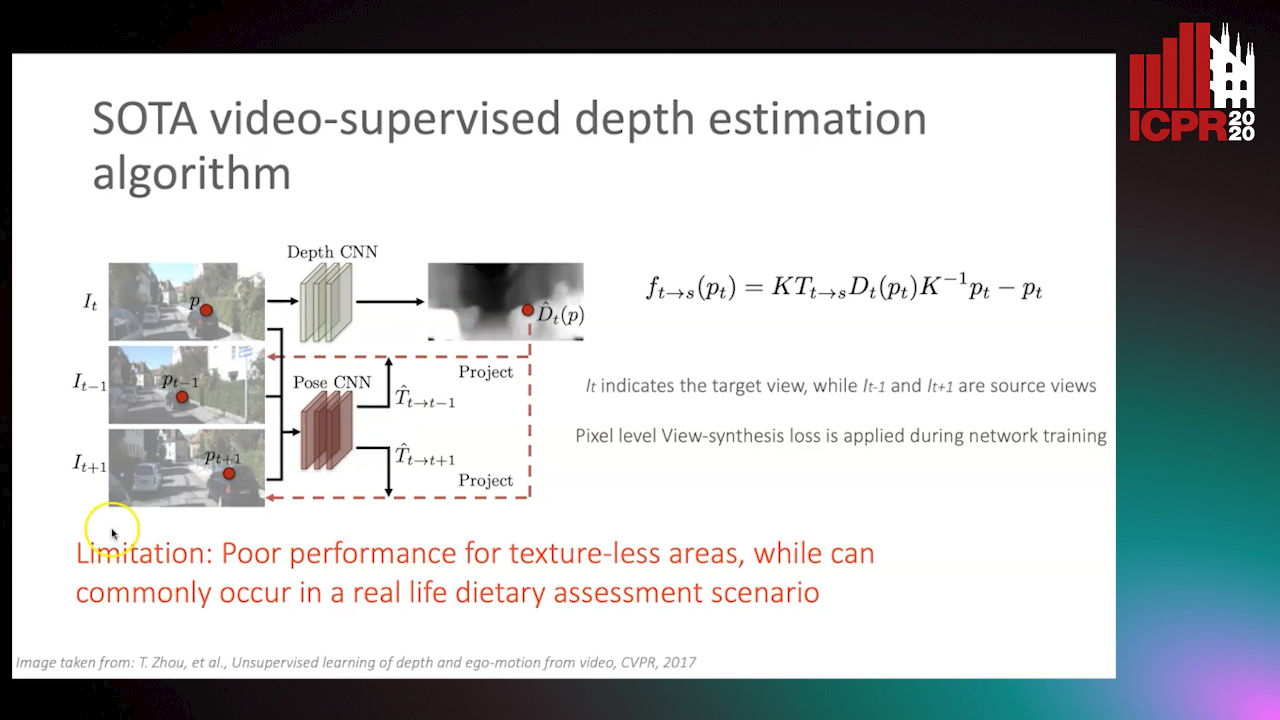
Auto-TLDR; Food Volume Estimation from a Single Food Image via Geometric Understanding and Semantic Prediction
Abstract Slides Poster Similar
Food volume estimation is an essential step in the pipeline of dietary assessment and demands the precise depth estimation of the food surface and table plane. Existing methods based on computer vision require either multi-image input or additional depth maps, reducing convenience of implementation and practical significance. Despite the recent advances in unsupervised depth estimation from a single image, the achieved performance in the case of large texture-less areas needs to be improved. In this paper, we propose a network architecture that jointly performs geometric understanding (i.e., depth prediction and 3D plane estimation) and semantic prediction on a single food image, enabling a robust and accurate food volume estimation regardless of the texture characteristics of the target plane. For the training of the network, only monocular videos with semantic ground truth are required, while the depth map and 3D plane ground truth are no longer needed. Experimental results on two separate food image databases demonstrate that our method performs robustly on texture-less scenarios and is superior to unsupervised networks and structure from motion based approaches, while it achieves comparable performance to fully-supervised methods.