Giancarlo Paoletti
Paper download is intended for registered attendees only, and is
subjected to the IEEE Copyright Policy. Any other use is strongly forbidden.
Papers from this author
Subspace Clustering for Action Recognition with Covariance Representations and Temporal Pruning
Giancarlo Paoletti, Jacopo Cavazza, Cigdem Beyan, Alessio Del Bue
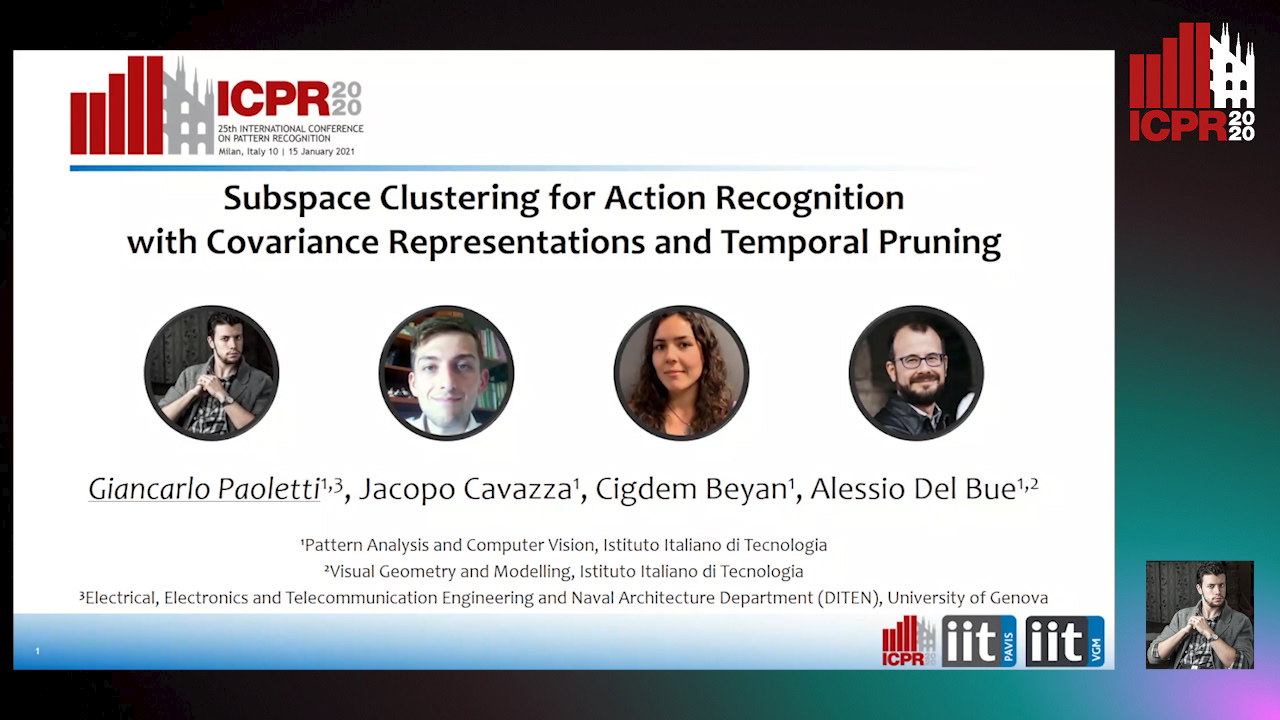
Auto-TLDR; Unsupervised Learning for Human Action Recognition from Skeletal Data
This paper tackles the problem of human action recognition, defined as classifying which action is displayed in a trimmed sequence, from skeletal data. Albeit state-of-the-art approaches designed for this application are all supervised, in this paper we pursue a more challenging direction: Solving the problem with unsupervised learning. To this end, we propose a novel subspace clustering method, which exploits covariance matrix to enhance the action’s discriminability and a timestamp pruning approach that allow us to better handle the temporal dimension of the data. Through a broad experimental validation, we show that our computational pipeline surpasses existing unsupervised approaches but also can result in favorable performances as compared to supervised methods.