Konstantinos Papadopoulos
Paper download is intended for registered attendees only, and is
subjected to the IEEE Copyright Policy. Any other use is strongly forbidden.
Papers from this author
Vertex Feature Encoding and Hierarchical Temporal Modeling in a Spatio-Temporal Graph Convolutional Network for Action Recognition
Konstantinos Papadopoulos, Enjie Ghorbel, Djamila Aouada, Bjorn Ottersten
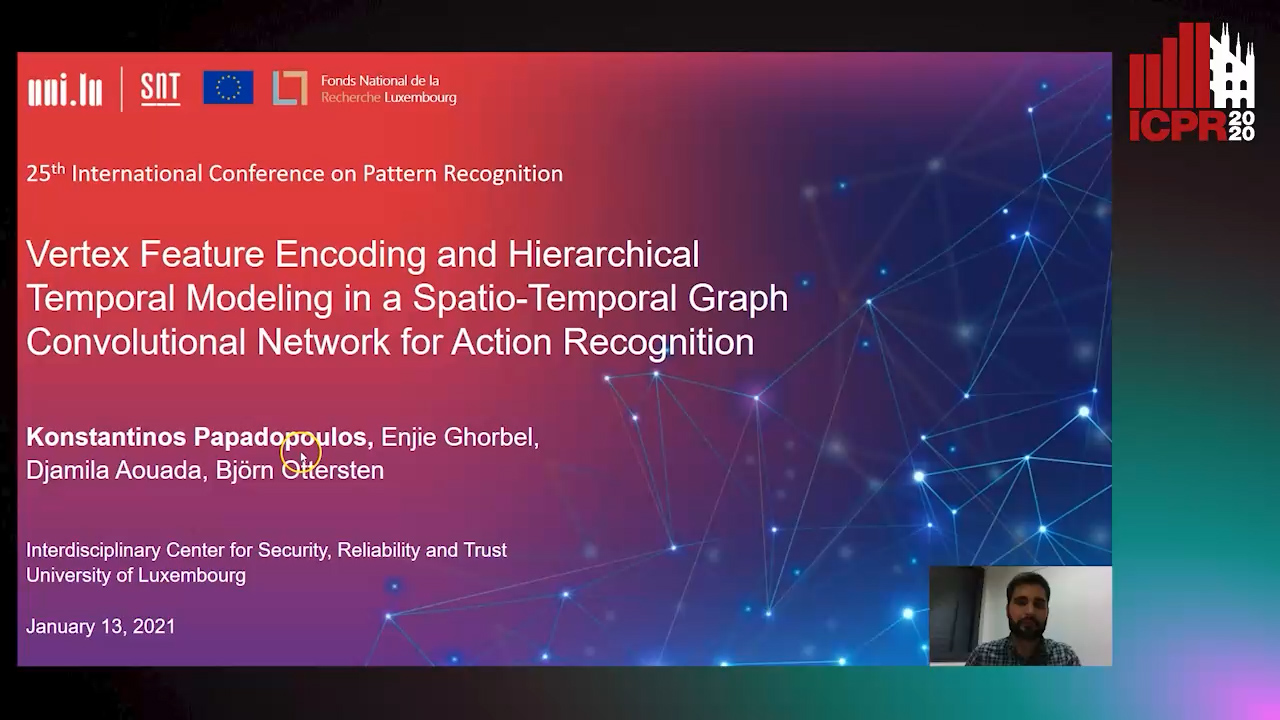
Auto-TLDR; Spatio-Temporal Graph Convolutional Network for Skeleton-Based Action Recognition
Abstract Slides Poster Similar
Spatio-temporal Graph Convolutional Networks (ST-GCNs) have shown great performance in the context of skeleton-based action recognition. Nevertheless, ST-GCNs use raw skeleton data as vertex features. Such features have low dimensionality and might not be optimal for action discrimination. Moreover, a single layer of temporal convolution is used to model short-term temporal dependencies but can be insufficient for capturing both long-term. In this paper, we extend the Spatio-Temporal Graph Convolutional Network for skeleton-based action recognition by introducing two novel modules, namely, the Graph Vertex Feature Encoder (GVFE) and the Dilated Hierarchical Temporal Convolutional Network (DH-TCN). On the one hand, the GVFE module learns appropriate vertex features for action recognition by encoding raw skeleton data into a new feature space. On the other hand, the DH-TCN module is capable of capturing both short-term and long-term temporal dependencies using a hierarchical dilated convolutional network. Experiments have been conducted on the challenging NTU RGB-D 60, NTU RGB-D 120 and Kinetics datasets. The obtained results show that our method competes with state-of-the-art approaches while using a smaller number of layers and parameters; thus reducing the required training time and memory.