Manasi Bharat Gund
Paper download is intended for registered attendees only, and is
subjected to the IEEE Copyright Policy. Any other use is strongly forbidden.
Papers from this author
Interpretable Emotion Classification Using Temporal Convolutional Models
Manasi Bharat Gund, Abhiram Ravi Bharadwaj, Ifeoma Nwogu
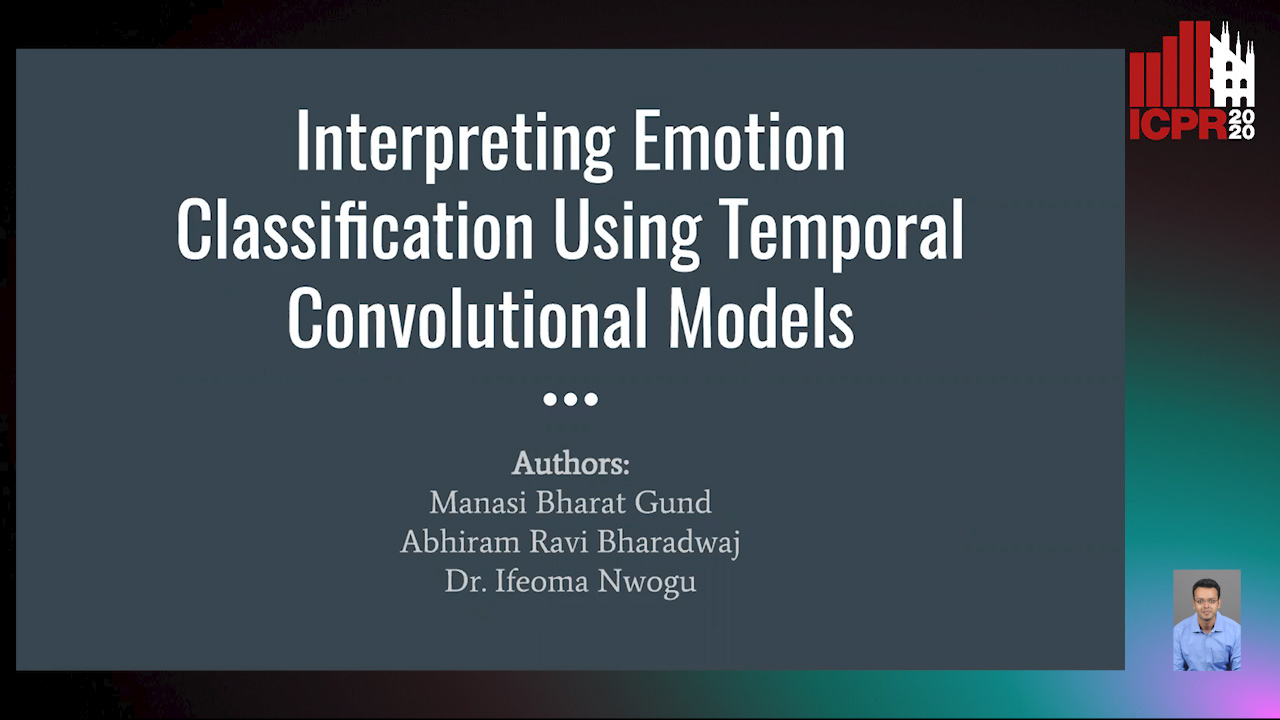
Auto-TLDR; Understanding the Dynamics of Facial Emotion Expression with Spatiotemporal Representations
Abstract Slides Poster Similar
As with many problems solved by deep neural networks, existing solutions rarely explain, precisely, the important factors responsible for the predictions made by the model. This work looks to investigate how different spatial regions and landmark points change in position over time, to better explain the underlying factors responsible for various facial emotion expressions. By pinpointing the specific regions or points responsible for the classification of a particular facial expression, we gain better insight into the dynamics of the face when displaying that emotion. To accomplish this, we examine two spatiotemporal representations of moving faces, while expressing different emotions. The representations are then presented to a convolutional neural network for emotion classification. Class activation maps are used in highlighting the regions of interest and the results are qualitatively compared with the well known facial action units, using the facial action coding system. The model was originally trained and tested on the CK+ dataset for emotion classification, and then generalized to the SAMM dataset. In so doing, we successfully present an interpretable technique for understanding the dynamics that occur during convolutional-based prediction tasks on sequences of face data.