Mirco Planamente
Paper download is intended for registered attendees only, and is
subjected to the IEEE Copyright Policy. Any other use is strongly forbidden.
Papers from this author
Self-Supervised Joint Encoding of Motion and Appearance for First Person Action Recognition
Mirco Planamente, Andrea Bottino, Barbara Caputo
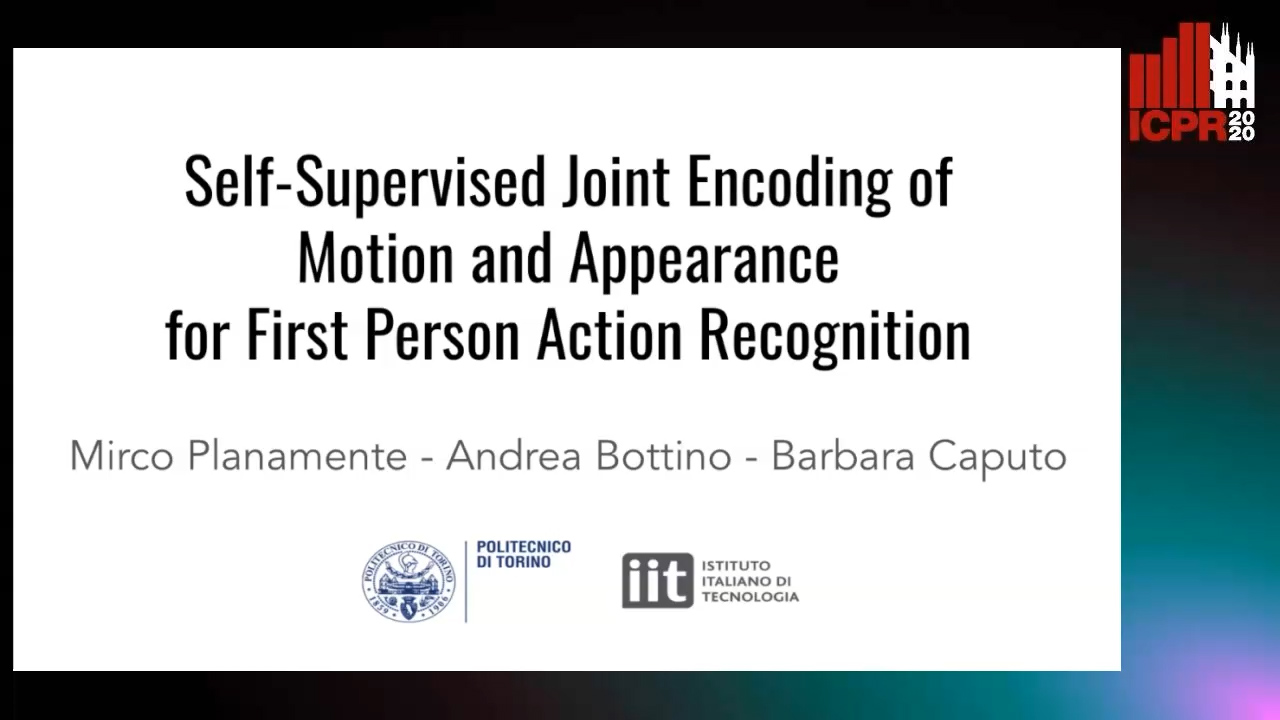
Auto-TLDR; A Single Stream Architecture for Egocentric Action Recognition from the First-Person Point of View
Abstract Slides Poster Similar
Wearable cameras are becoming more and more popular in several applications, increasing the interest of the research community in developing approaches for recognizing actions from the first-person point of view. An open challenge in egocentric action recognition is that videos lack detailed information about the main actor's pose and thus tend to record only parts of the movement when focusing on manipulation tasks. Thus, the amount of information about the action itself is limited, making crucial the understanding of the manipulated objects and their context. Many previous works addressed this issue with two-stream architectures, where one stream is dedicated to modeling the appearance of objects involved in the action, and another to extracting motion features from optical flow. In this paper, we argue that learning features jointly from these two information channels is beneficial to capture the spatio-temporal correlations between the two better. To this end, we propose a single stream architecture able to do so, thanks to the addition of a self-supervised block that uses a pretext motion prediction task to intertwine motion and appearance knowledge. Experiments on several publicly available databases show the power of our approach.