Peixian Hong
Paper download is intended for registered attendees only, and is
subjected to the IEEE Copyright Policy. Any other use is strongly forbidden.
Papers from this author
Semi-Supervised Person Re-Identification by Attribute Similarity Guidance
Peixian Hong, Ancong Wu, Wei-Shi Zheng
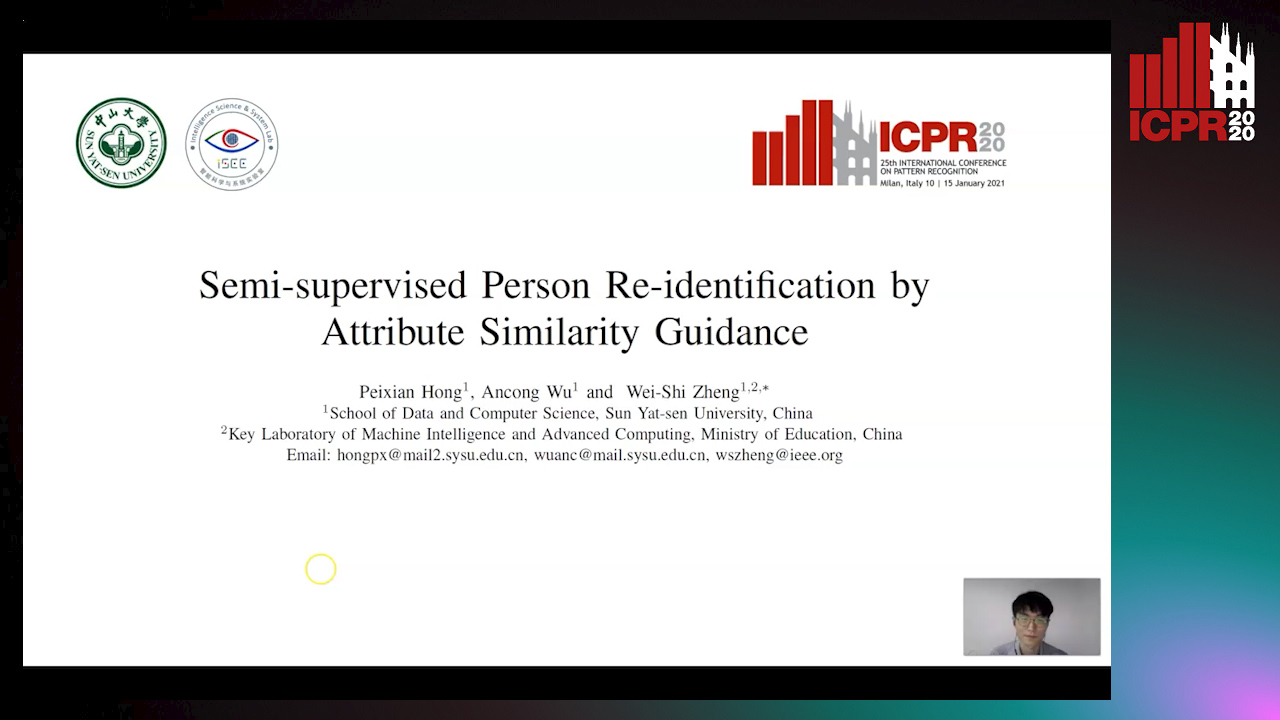
Auto-TLDR; Attribute Similarity Guidance Guidance Loss for Semi-supervised Person Re-identification
Abstract Slides Poster Similar
Although supervised person re-identification (RE-ID) has achieved great progress with deep learning, it requires time-consuming annotation of a large number of pedestrian identities. To reduce labeling cost, we attempt to reduce cross-camera identity annotations and exploit pedestrian attribute annotations as auxiliary information instead. The pedestrian attributes, such as outfit styles, contain coarse semantic knowledge. Although pedestrian attributes are annotated without exhaustive searching in a camera network, which is much easier than cross-camera identity annotation, ambiguity exists in attributes when different persons have similar outfits. To solve this problem, we propose an Attribute Similarity Guidance loss (ASG) to guide appearance feature learning for RE-ID by selective attribute similarity preservation to avoid the impact of such ambiguity. Finally, we develop an attribute-guided self training framework to jointly utilize attribute annotations, unlabeled data and limited labeled data for semi-supervised learning. Extensive experiments on Market-1501 and DukeMTMC-ReID show the superiority of our method for semi-supervised RE-ID.