Bo Hu
Paper download is intended for registered attendees only, and is
subjected to the IEEE Copyright Policy. Any other use is strongly forbidden.
Papers from this author
Energy-Constrained Self-Training for Unsupervised Domain Adaptation
Xiaofeng Liu, Xiongchang Liu, Bo Hu, Jun Lu, Jonghye Woo, Jane You
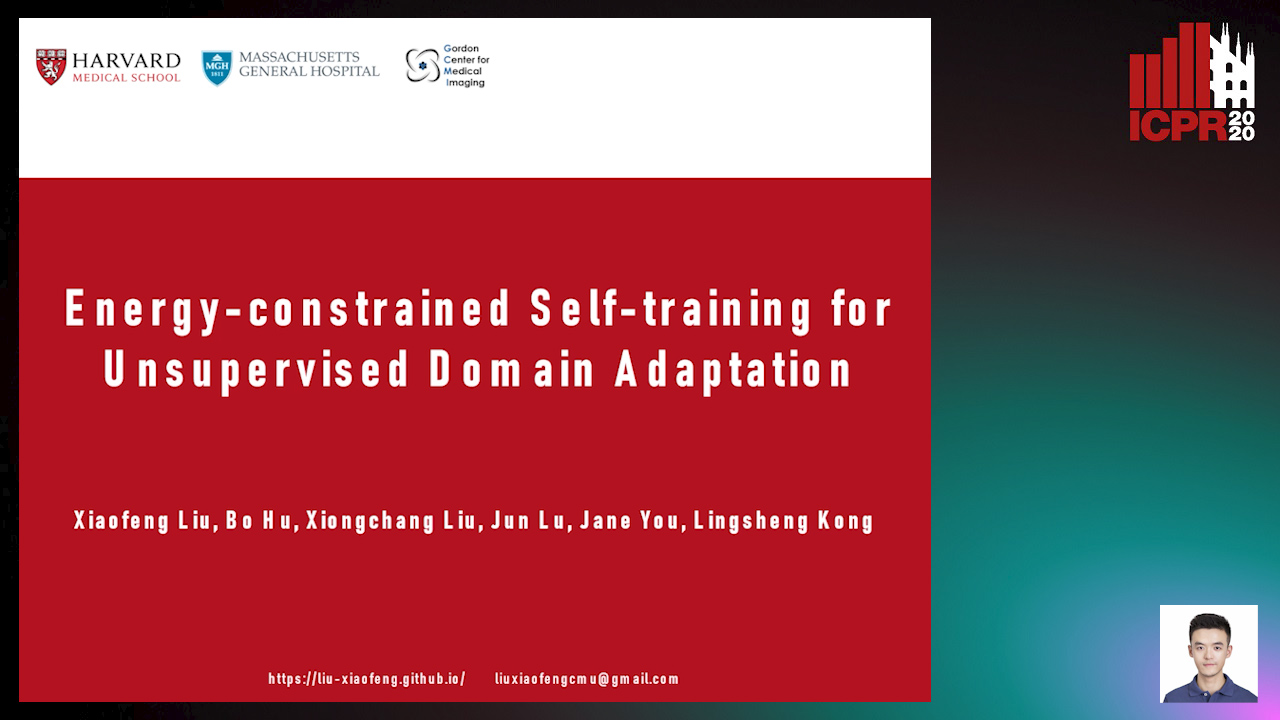
Auto-TLDR; Unsupervised Domain Adaptation with Energy Function Minimization
Abstract Slides Poster Similar
Unsupervised domain adaptation (UDA) aims to transfer the knowledge on a labeled source domain distribution to perform well on an unlabeled target domain. Recently, the deep self-training involves an iterative process of predicting on the target domain and then taking the confident predictions as hard pseudo-labels for retraining. However, the pseudo-labels are usually unreliable, and easily leading to deviated solutions with propagated errors. In this paper, we resort to the energy-based model and constrain the training of the unlabeled target sample with the energy function minimization objective. It can be applied as a simple additional regularization. In this framework, it is possible to gain the benefits of the energy-based model, while retaining strong discriminative performance following a plug-and-play fashion. The convergence property and its connection with classification expectation minimization are investigated. We deliver extensive experiments on the most popular and large scale UDA benchmarks of image classification as well as semantic segmentation to demonstrate its generality and effectiveness.