Ziqi Wang
Paper download is intended for registered attendees only, and is
subjected to the IEEE Copyright Policy. Any other use is strongly forbidden.
Papers from this author
Respecting Domain Relations: Hypothesis Invariance for Domain Generalization
Ziqi Wang, Marco Loog, Jan Van Gemert
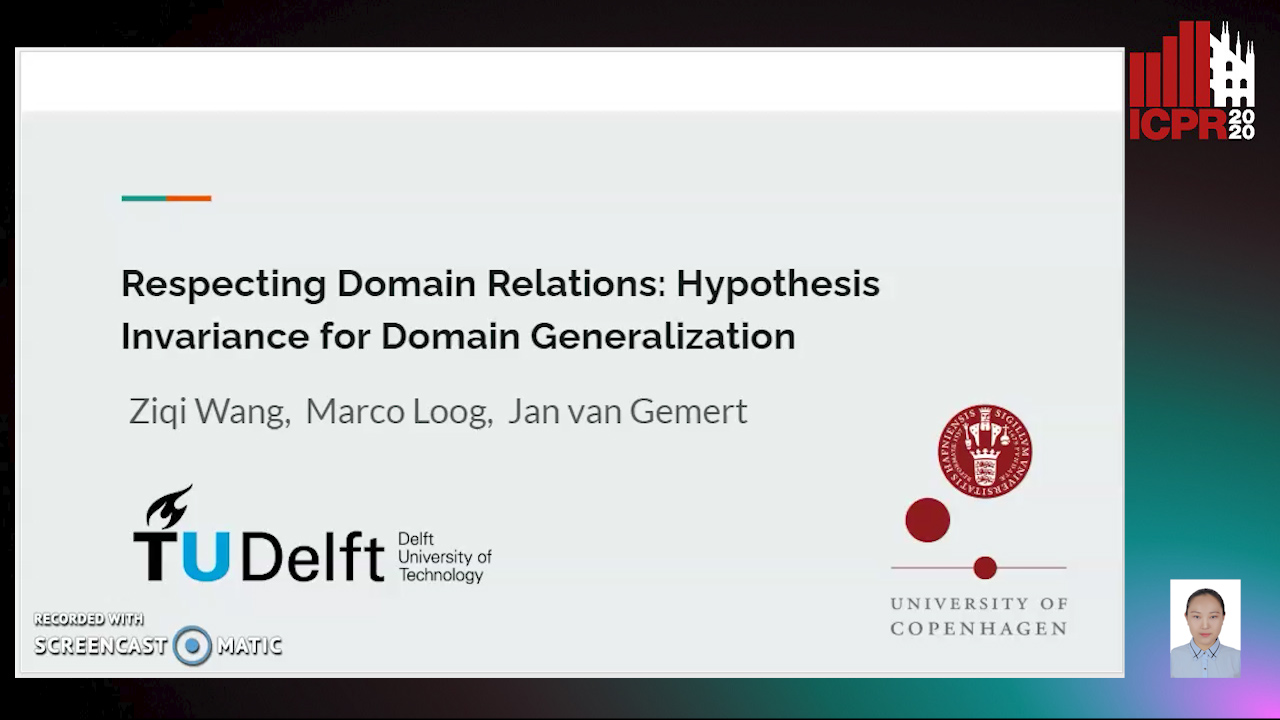
Auto-TLDR; Learning Hypothesis Invariant Representations for Domain Generalization
Abstract Slides Poster Similar
In domain generalization, multiple labeled non-independent and non-identically distributed source domains are available during training while neither the data nor the labels of target domains are. Currently, learning so-called domain invariant representations (DIRs) is the prevalent approach to domain generalization. In this work, we define DIRs employed by existing works in probabilistic terms and show that by learning DIRs, overly strict requirements are imposed concerning the invariance. Particularly, DIRs aim to perfectly align representations of different domains, i.e. their input distributions. This is, however, not necessary for good generalization to a target domain and may even dispose of valuable classification information. We propose to learn so-called hypothesis invariant representations (HIRs), which relax the invariance assumptions. We report experimental results on public domain generalization datasets to show that learning HIRs is more effective than learning DIRs. In fact, our approach can even compete with approaches using prior knowledge about domains.