Chaoyi Hong
Paper download is intended for registered attendees only, and is
subjected to the IEEE Copyright Policy. Any other use is strongly forbidden.
Papers from this author
Parallel Network to Learn Novelty from the Known
Shuaiyuan Du, Chaoyi Hong, Zhiyu Pan, Chen Feng, Zhiguo Cao
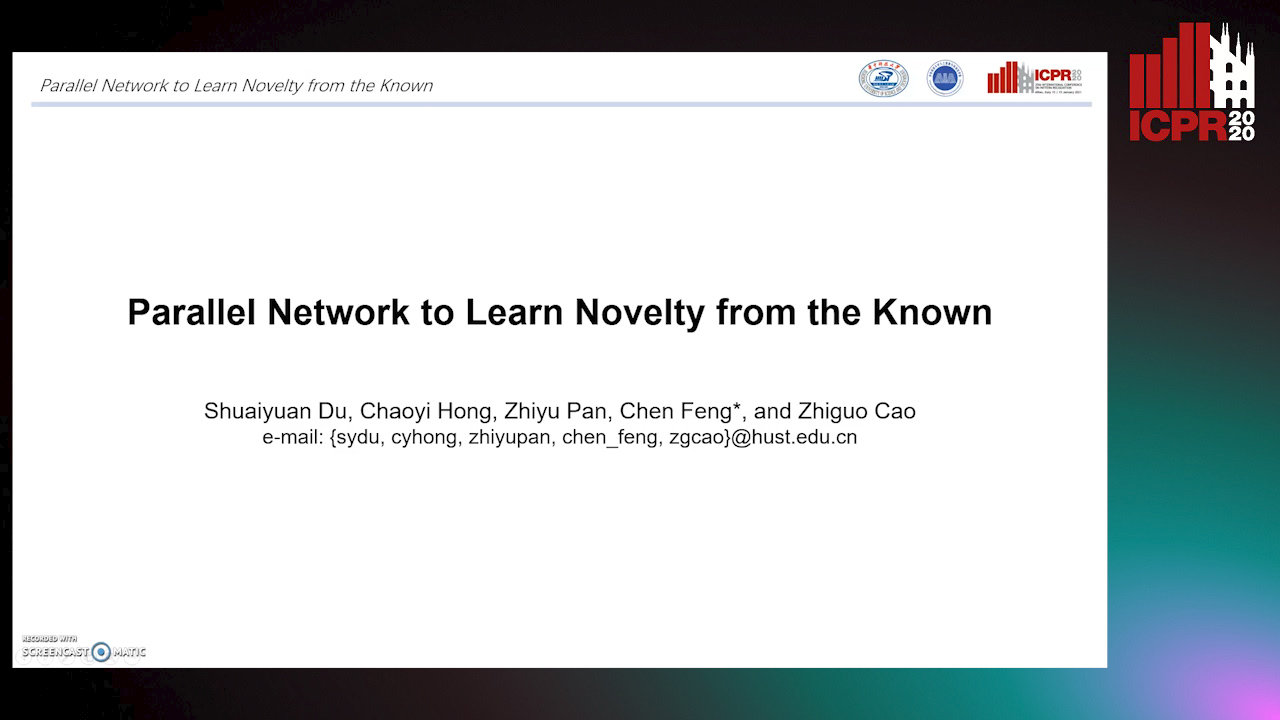
Auto-TLDR; Trainable Parallel Network for Pseudo-Novel Detection
Abstract Slides Poster Similar
Towards multi-class novelty detection, we propose an end-to-end trainable Parallel Network (PN) using no additional data but only the training set itself. Our key idea is to first divide the training set into successive subtasks of pseudo-novelty detection to simulate real scenarios. We then design a multi-branch PN to well address the fine-grained division, which yields a compressed and more discriminative classification space and forms a natural ensemble. In practice, we divide the training data into subsets consisting of known and pseudo-novel classes. Each subset forms a sub-task fed to one branch in PN. During training, both known and pseudo-novel classes are uniformly distributed over the branches for better data balance and model diversity. By distinguishing between the known and the diverse pseudo-novel, PN extracts the concept of novelty in a compressed classification space. This provides PN with generalization ability to real novel classes which are absent during training. During online inference, this ability is further strengthened with the ensemble of PN's multiple branches. Experiments on three public datasets show our method's superiority to the mainstream methods.