Andrea Palazzi
Paper download is intended for registered attendees only, and is
subjected to the IEEE Copyright Policy. Any other use is strongly forbidden.
Papers from this author
Future Urban Scenes Generation through Vehicles Synthesis
Alessandro Simoni, Luca Bergamini, Andrea Palazzi, Simone Calderara, Rita Cucchiara
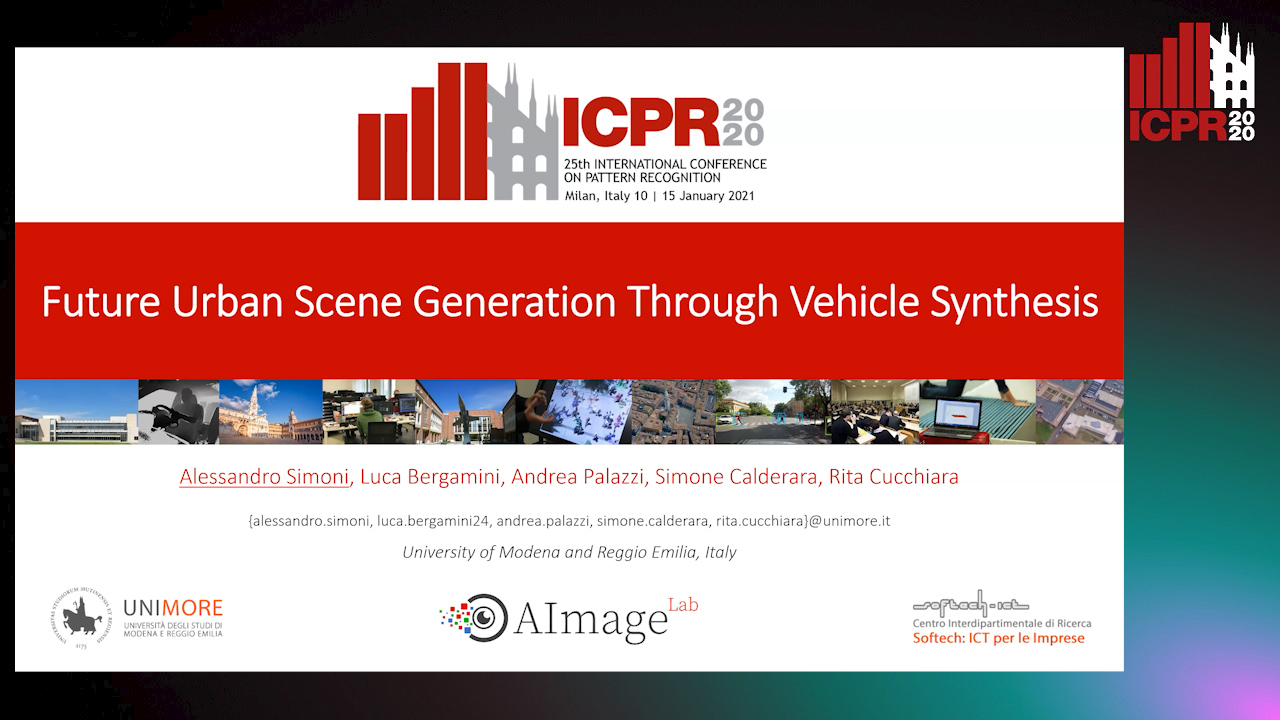
Auto-TLDR; Predicting the Future of an Urban Scene with a Novel View Synthesis Paradigm
Abstract Slides Poster Similar
In this work we propose a deep learning pipeline to predict the visual future appearance of an urban scene. Despite recent advances, generating the entire scene in an end-to-end fashion is still far from being achieved. Instead, here we follow a two stages approach, where interpretable information is included in the loop and each actor is modelled independently. We leverage a per-object novel view synthesis paradigm; i.e. generating a synthetic representation of an object undergoing a geometrical roto-translation in the 3D space. Our model can be easily conditioned with constraints (e.g. input trajectories) provided by state-of-the-art tracking methods or by the user itself. This allows us to generate a set of diverse realistic futures starting from the same input in a multi-modal fashion. We visually and quantitatively show the superiority of this approach over traditional end-to-end scene-generation methods on CityFlow, a challenging real world dataset.