Feng-Guang Su
Paper download is intended for registered attendees only, and is
subjected to the IEEE Copyright Policy. Any other use is strongly forbidden.
Papers from this author
Learning Interpretable Representation for 3D Point Clouds
Feng-Guang Su, Ci-Siang Lin, Yu-Chiang Frank Wang
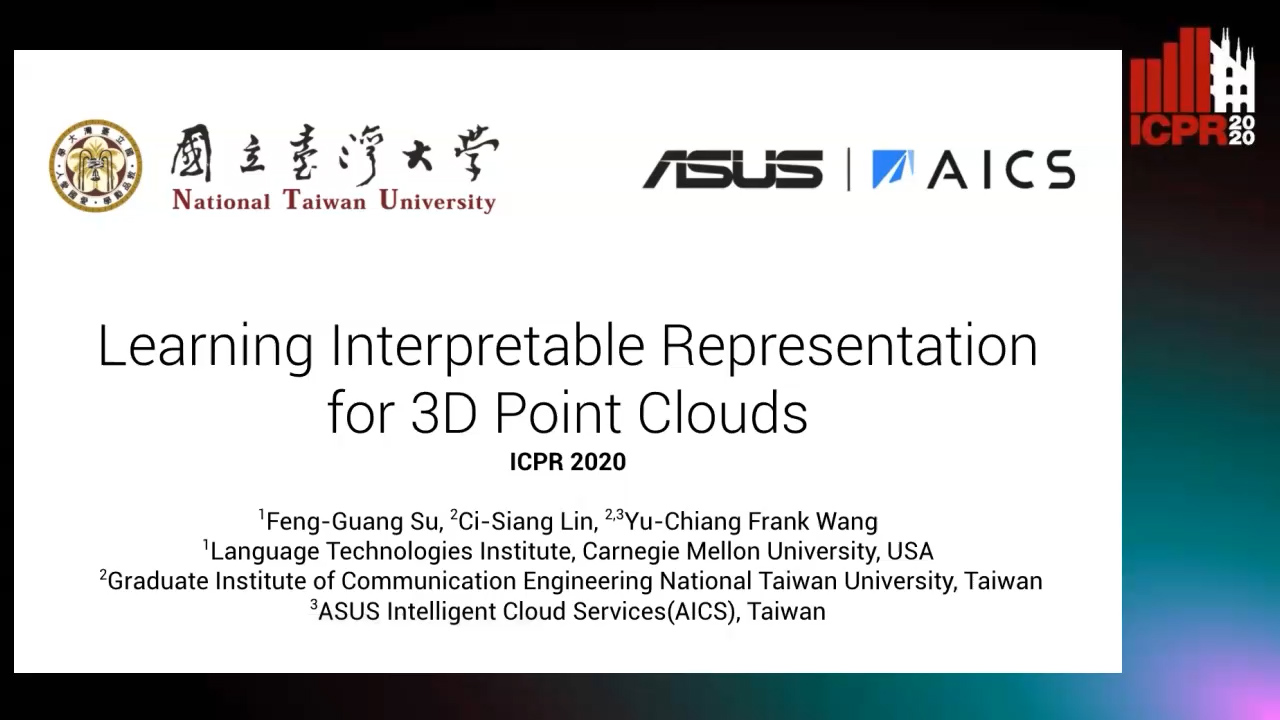
Auto-TLDR; Disentangling Body-type and Pose Information from 3D Point Clouds Using Adversarial Learning
Abstract Slides Poster Similar
Point clouds have emerged as a popular representation of 3D visual data. With a set of unordered 3D points, one typically needs to transform them into latent representation before further classification and segmentation tasks. However, one cannot easily interpret such encoded latent representation. To address this issue, we propose a unique deep learning framework for disentangling body-type and pose information from 3D point clouds. Extending from autoenoder, we advance adversarial learning a selected feature type, while classification and data recovery can be additionally observed. Our experiments confirm that our model can be successfully applied to perform a wide range of 3D applications like shape synthesis, action translation, shape/action interpolation, and synchronization.