Ayako Amma
Paper download is intended for registered attendees only, and is
subjected to the IEEE Copyright Policy. Any other use is strongly forbidden.
Papers from this author
Median-Shape Representation Learning for Category-Level Object Pose Estimation in Cluttered Environments
Hiroki Tatemichi, Yasutomo Kawanishi, Daisuke Deguchi, Ichiro Ide, Hiroshi Murase, Ayako Amma
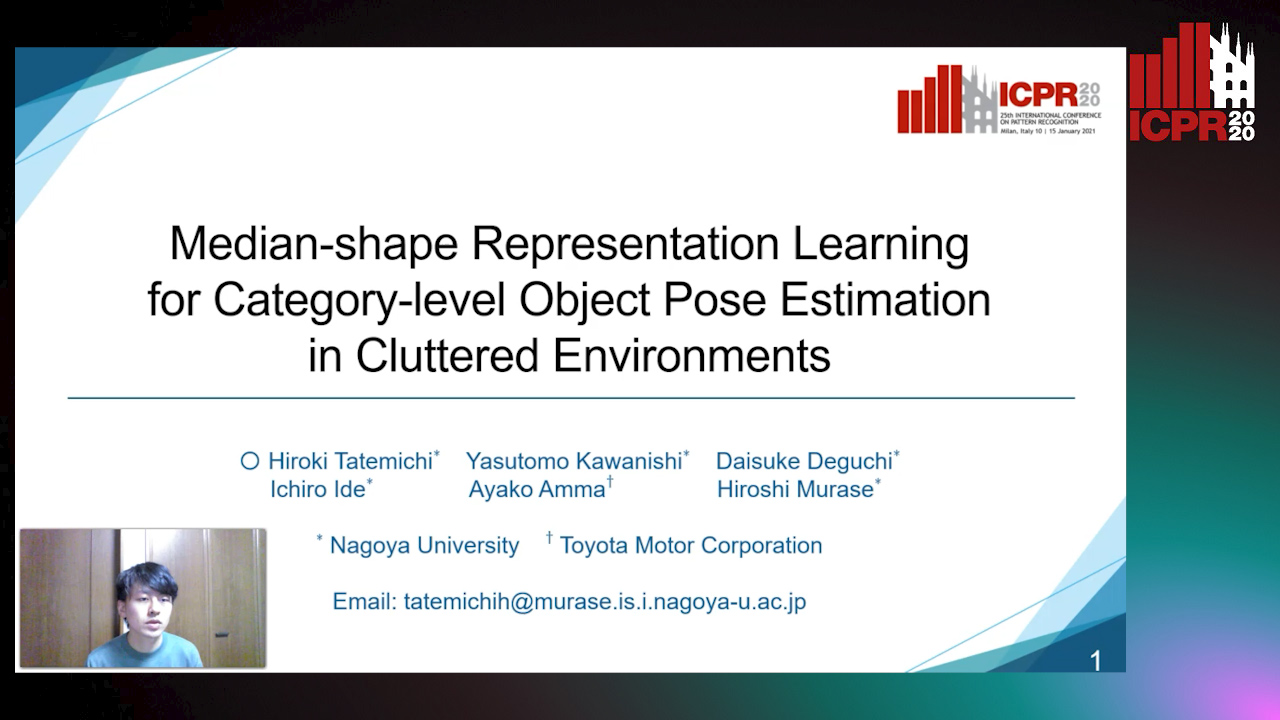
Auto-TLDR; An Occlusion-Robust Pose Estimation Method from a Depth Image
Abstract Slides Poster Similar
In this paper, we propose an occlusion-robust pose estimation method of an unknown object instance in an object category from a depth image. In a cluttered environment, objects are often occluded mutually. For estimating the pose of an object in such a situation, a method that de-occludes the unobservable area of the object would be effective. However, there are two difficulties; occlusion causes offset between the actual object center and the center of its observable area, and different instances in a category may have different shapes. To cope with these difficulties, we propose a two-stage Encoder-Decoder model to extract features with objects whose centers are aligned to the image center. In the model, we also propose the Median-shape Reconstructor as the second stage to absorb shape variations in a category. By evaluating the method with both a large-scale virtual dataset and a real dataset, we confirmed the proposed method achieves good performance on pose estimation of an occluded object from a depth image.