Jing Liu
Paper download is intended for registered attendees only, and is
subjected to the IEEE Copyright Policy. Any other use is strongly forbidden.
Papers from this author
Multi-Scale Residual Pyramid Attention Network for Monocular Depth Estimation
Jing Liu, Xiaona Zhang, Zhaoxin Li, Tianlu Mao
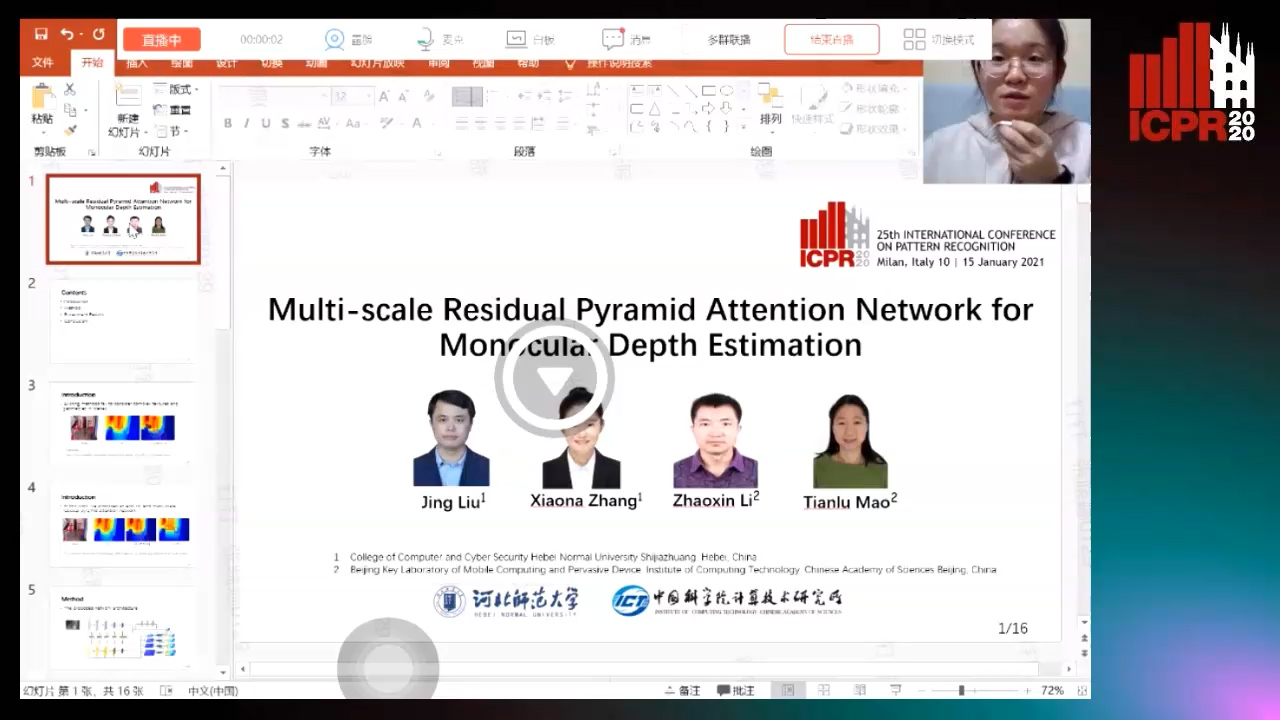
Auto-TLDR; Multi-scale Residual Pyramid Attention Network for Monocular Depth Estimation
Abstract Slides Poster Similar
Monocular depth estimation is a challenging problem in computer vision and is crucial for understanding 3D scene geometry. Recently, deep convolutional neural networks (DCNNs) based methods have improved the estimation accuracy significantly. However, existing methods fail to consider complex textures and geometries in scenes, thereby resulting in loss of local details, distorted object boundaries, and blurry reconstruction. In this paper, we proposed an end-to-end Multi-scale Residual Pyramid Attention Network (MRPAN) to mitigate these problems.First,we propose a Multi-scale Attention Context Aggregation (MACA) module, which consists of Spatial Attention Module (SAM) and Global Attention Module (GAM). By considering the position and scale correlation of pixels from spatial and global perspectives, the proposed module can adaptively learn the similarity between pixels so as to obtain more global context information of the image and recover the complex structure in the scene. Then we proposed an improved Residual Refinement Module (RRM) to further refine the scene structure, giving rise to deeper semantic information and retain more local details. Experimental results show that our method achieves more promisin performance in object boundaries and local details compared with other state-of-the-art methods.