Chengxin Liu
Paper download is intended for registered attendees only, and is
subjected to the IEEE Copyright Policy. Any other use is strongly forbidden.
Papers from this author
Exploiting Distilled Learning for Deep Siamese Tracking
Chengxin Liu, Zhiguo Cao, Wei Li, Yang Xiao, Shuaiyuan Du, Angfan Zhu
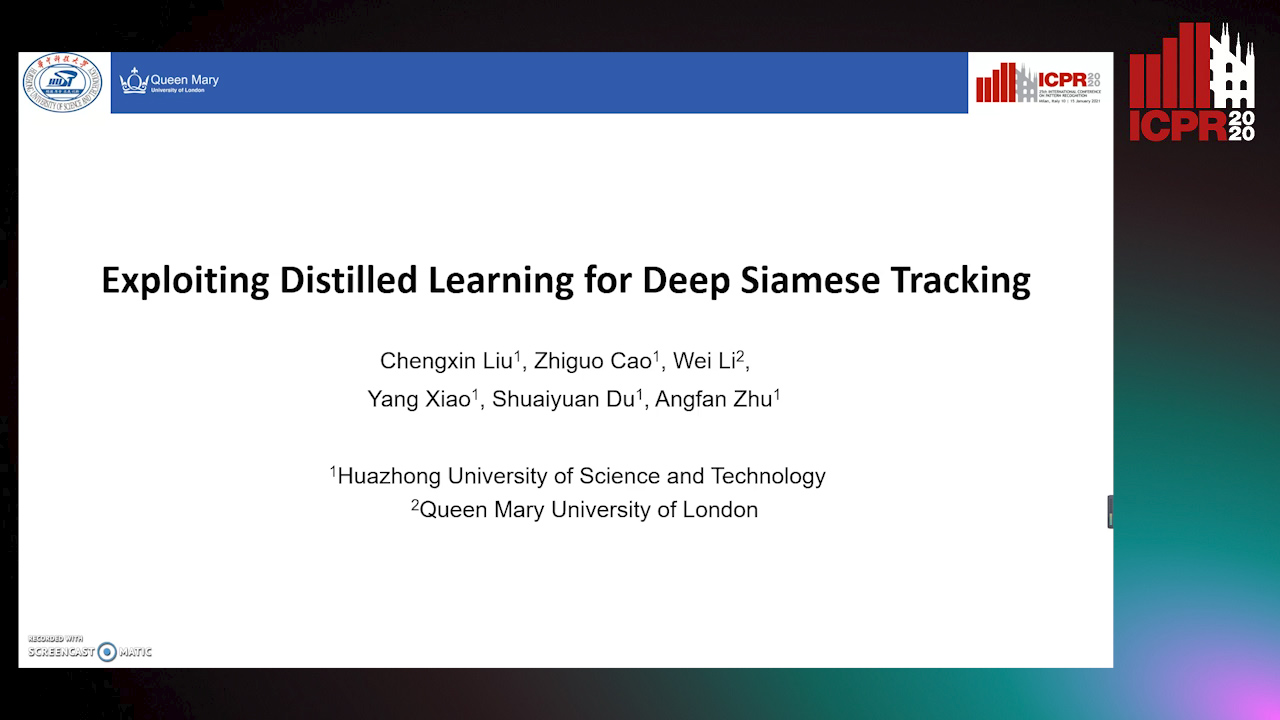
Auto-TLDR; Distilled Learning Framework for Siamese Tracking
Abstract Slides Poster Similar
Existing deep siamese trackers are typically built on off-the-shelf CNN models for feature learning, with the demand for huge power consumption and memory storage. This limits current deep siamese trackers to be carried on resource-constrained devices like mobile phones, given factor that such a deployment normally requires cost-effective considerations. In this work, we address this issue by presenting a novel Distilled Learning Framework(DLF) for siamese tracking, which aims at learning tracking model with efficiency and high accuracy. Specifically, we propose two simple yet effective knowledge distillation strategies, denote as point-wise distillation and pair-wise distillation, which are designed for transferring knowledge from a more discriminative teacher tracker into a compact student tracker. In this way, cost-effective and high performance tracking could be achieved. Extensive experiments on several tracking benchmarks demonstrate the effectiveness of our proposed method.