Yang Fang
Paper download is intended for registered attendees only, and is
subjected to the IEEE Copyright Policy. Any other use is strongly forbidden.
Papers from this author
RSINet: Rotation-Scale Invariant Network for Online Visual Tracking
Yang Fang, Geunsik Jo, Chang-Hee Lee
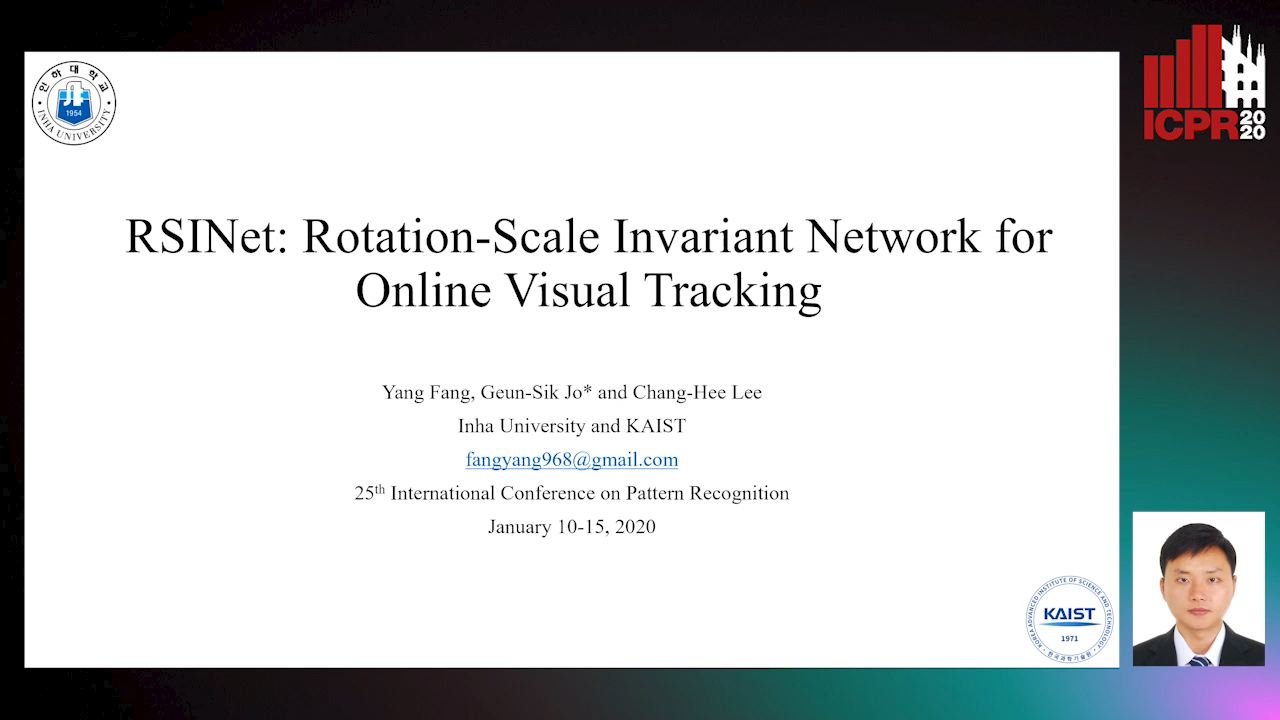
Auto-TLDR; RSINet: Rotation-Scale Invariant Network for Adaptive Tracking
Abstract Slides Poster Similar
Most Siamese network-based trackers perform the tracking process without model update, and cannot learn target-specific variation adaptively. Moreover, Siamese-based trackers infer the new state of tracked objects by generating axis-aligned bounding boxes, which contain extra background noise, and are unable to accurately estimate the rotation and scale transformation of moving objects, thus potentially reducing tracking performance. In this paper, we propose a novel Rotation-Scale Invariant Network (RSINet) to address the above problem. Our RSINet tracker consists of a target-distractor discrimination branch and a rotation-scale estimation branch, the rotation and scale knowledge can be explicitly learned by a multi-task learning method in an end-to-end manner. In addtion, the tracking model is adaptively optimized and updated under spatio-temporal energy control, which ensures model stability and reliability, as well as high tracking efficiency. Comprehensive experiments on OTB-100, VOT2018, and LaSOT benchmarks demonstrate that our proposed RSINet tracker yields new state-of-the-art performance compared with recent trackers, while running at real-time speed about 45 FPS.