Brian Kostadinov Shalon Isaac-Medina
Paper download is intended for registered attendees only, and is
subjected to the IEEE Copyright Policy. Any other use is strongly forbidden.
Papers from this author
Multi-View Object Detection Using Epipolar Constraints within Cluttered X-Ray Security Imagery
Brian Kostadinov Shalon Isaac-Medina, Chris G. Willcocks, Toby Breckon
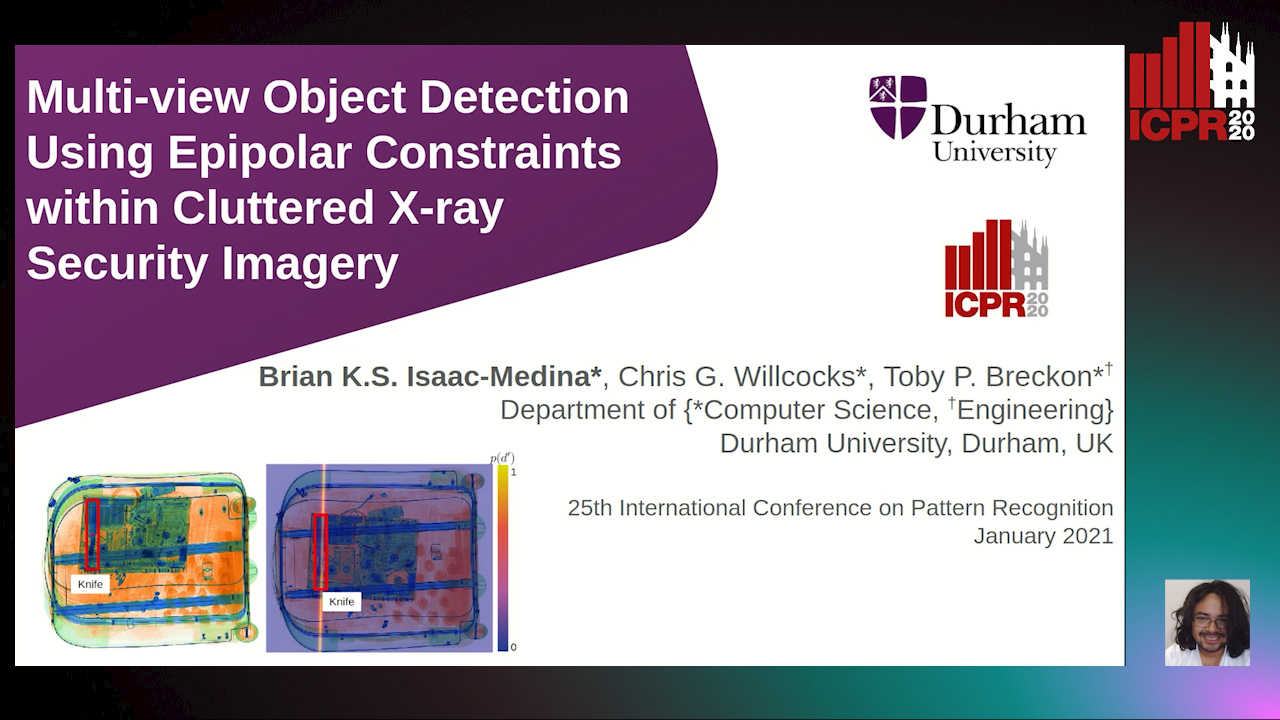
Auto-TLDR; Exploiting Epipolar Constraints for Multi-View Object Detection in X-ray Security Images
Abstract Slides Poster Similar
Automatic detection for threat object items is an increasing emerging area of future application in X-ray security imagery. Although modern X-ray security scanners can provide two or more views, the integration of such object detectors across the views has not been widely explored with rigour. Therefore, we investigate the application of geometric constraints using the epipolar nature of multi-view imagery to improve object detection performance. Furthermore, we assume that images come from uncalibrated views, such that a method to estimate the fundamental matrix using ground truth bounding box centroids from multiple view object detection labels is proposed. In addition, detections are given a score based on its similarity with respect to the distribution of the error of the epipolar estimation. This score is used as confidence weights for merging duplicated predictions using non-maximum suppression. Using a standard object detector (YOLOv3), our technique increases the average precision of detection by 2.8% on a dataset composed of firearms, laptops, knives and cameras. These results indicate that the integration of images at different views significantly improves the detection performance of threat items of cluttered X-ray security images.