Eleonora Vig
Paper download is intended for registered attendees only, and is
subjected to the IEEE Copyright Policy. Any other use is strongly forbidden.
Papers from this author
Aerial Road Segmentation in the Presence of Topological Label Noise
Corentin Henry, Friedrich Fraundorfer, Eleonora Vig
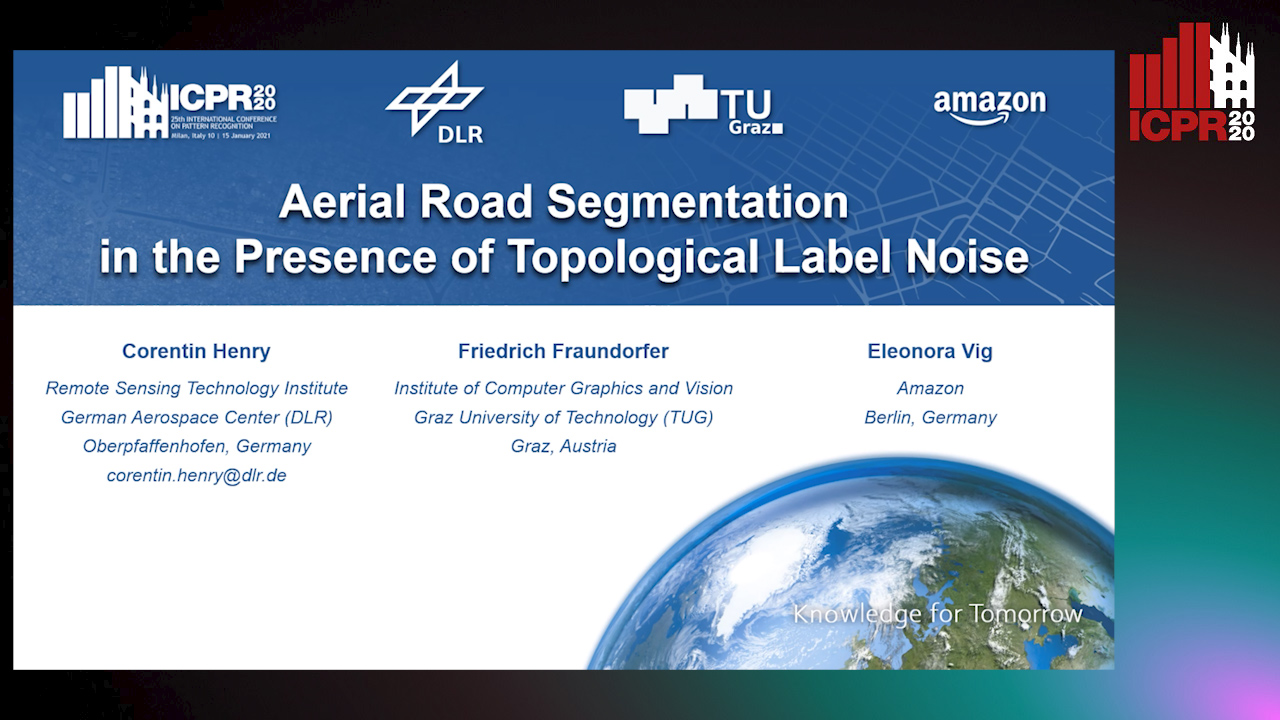
Auto-TLDR; Improving Road Segmentation with Noise-Aware U-Nets for Fine-Grained Topology delineation
Abstract Slides Poster Similar
The availability of large-scale annotated datasets has enabled Fully-Convolutional Neural Networks to reach outstanding performance on road extraction in aerial images. However, high-quality pixel-level annotation is expensive to produce and even manually labeled data often contains topological errors. Trading off quality for quantity, many datasets rely on already available yet noisy labels, for example from OpenStreetMap. In this paper, we explore the training of custom U-Nets built with ResNet and DenseNet backbones using noise-aware losses that are robust towards label omission and registration noise. We perform an extensive evaluation of standard and noise-aware losses, including a novel Bootstrapped DICE-Coefficient loss, on two challenging road segmentation benchmarks. Our losses yield a consistent improvement in overall extraction quality and exhibit a strong capacity to cope with severe label noise. Our method generalizes well to two other fine-grained topology delineation tasks: surface crack detection for quality inspection and cell membrane extraction in electron microscopy imagery.