Jukka Peltomäki
Paper download is intended for registered attendees only, and is
subjected to the IEEE Copyright Policy. Any other use is strongly forbidden.
Papers from this author
Loop-closure detection by LiDAR scan re-identification
Jukka Peltomäki, Xingyang Ni, Jussi Puura, Joni-Kristian Kamarainen, Heikki Juhani Huttunen
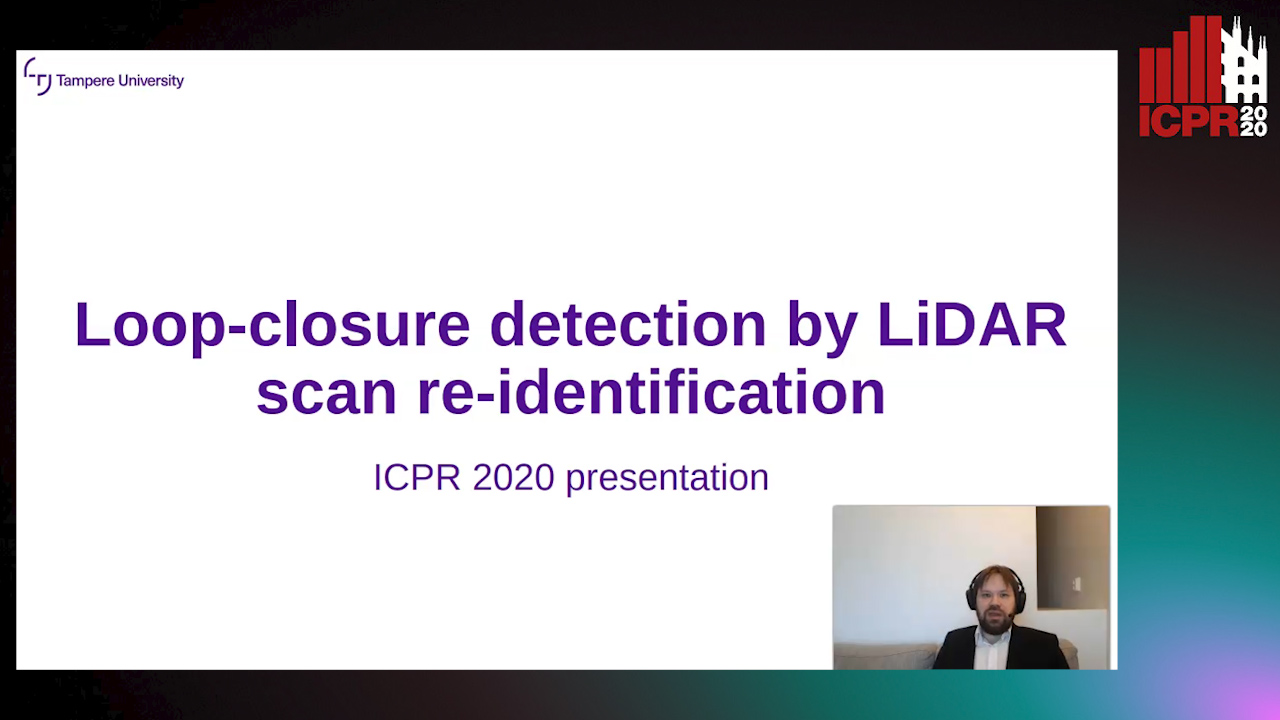
Auto-TLDR; Loop-Closing Detection from LiDAR Scans Using Convolutional Neural Networks
Abstract Slides Poster Similar
In this work, loop-closure detection from LiDAR scans is defined as an image re-identification problem. Re-identification is performed by computing Euclidean distances of a query scan to a gallery set of previous scans. The distances are computed in a feature embedding space where the scans are mapped by a convolutional neural network (CNN). The network is trained using the triplet loss training strategy. In our experiments we compare different backbone networks, variants of the triplet loss and generic and LiDAR specific data augmentation techniques. With a realistic indoor dataset the best architecture obtains the mean average precision (mAP) above 90%.