Yuanhang Ren
Paper download is intended for registered attendees only, and is
subjected to the IEEE Copyright Policy. Any other use is strongly forbidden.
Papers from this author
Uniform and Non-Uniform Sampling Methods for Sub-Linear Time K-Means Clustering
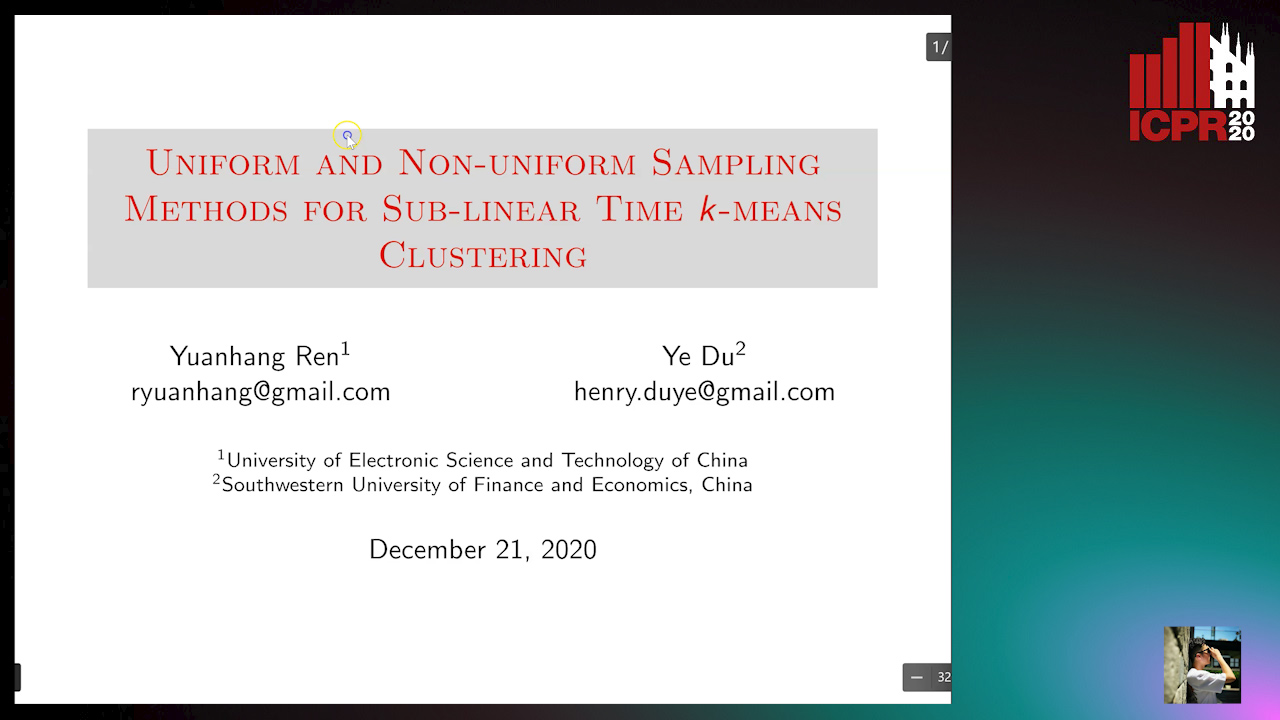
Auto-TLDR; Sub-linear Time Clustering with Constant Approximation Ratio for K-Means Problem
Abstract Slides Poster Similar
The $k$-means problem is arguably the most well-known clustering problem in machine learning, and lots of approximation algorithms have been proposed for it. However, many of these algorithms may become infeasible when data is huge. Sub-linear time algorithms with constant approximation ratios are desirable in this scenario. In this paper, we first improve the analysis of the algorithm proposed by \cite{Mohan:2017:BNA:3172077.3172235} by sharpening the approximation ratio from $4(\alpha+\beta)$ to $\alpha+\beta$. Moreover, on mild assumptions of the data, a constant approximation ratio can be achieved in poly-logarithmic time by the algorithm. Furthermore, we propose a novel sub-linear time clustering algorithm called {\it Double-K-M$\text{C}^2$ sampling} as well. Experiments on the data clustering task and the image segmentation task have validated the effectiveness of our algorithms.