Masaki Nakada
Paper download is intended for registered attendees only, and is
subjected to the IEEE Copyright Policy. Any other use is strongly forbidden.
Papers from this author
Locally-Connected, Irregular Deep Neural Networks for Biomimetic Active Vision in a Simulated Human
Masaki Nakada, Honglin Chen, Arjun Lakshmipathy, Demetri Terzopoulos
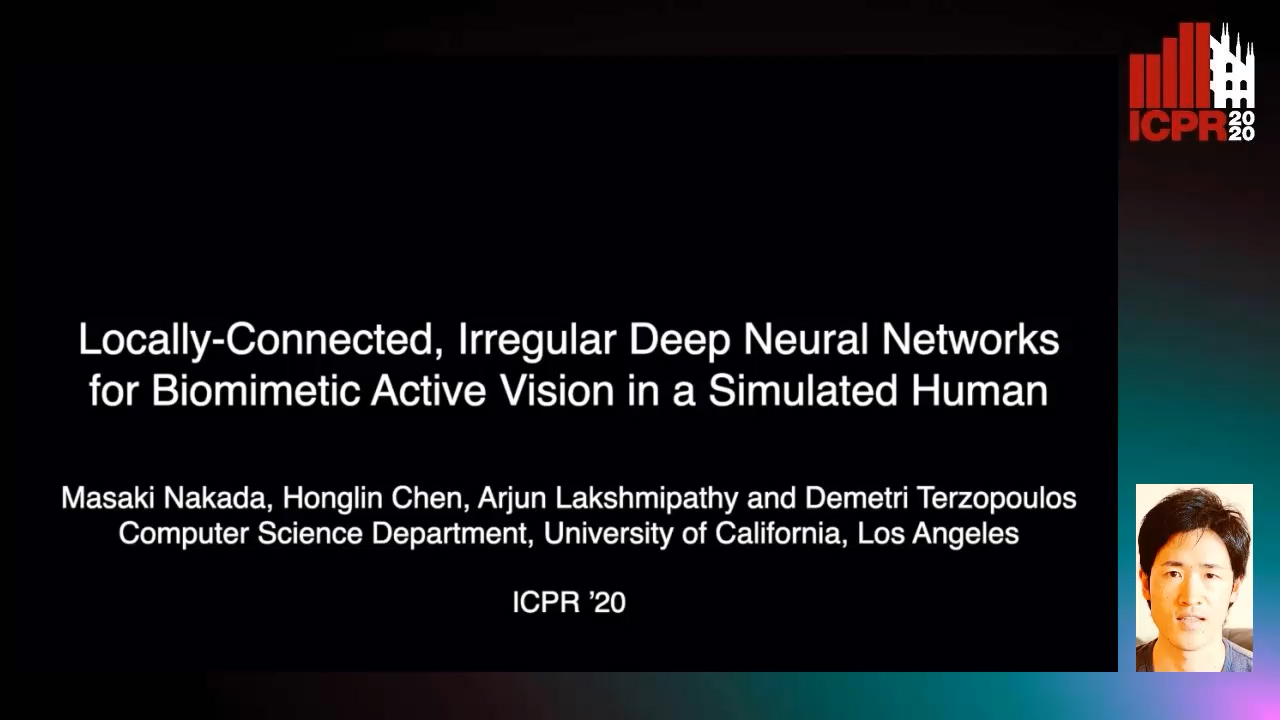
Auto-TLDR; Local-connected, Irregular Deep Neural Networks for biomimetic active vision
Abstract Slides Poster Similar
An advanced simulation framework has recently been introduced for exploring human perception and visuomotor control. In this context, we investigate locally-connected, irregular deep neural networks (liNets) for biomimetic active vision. Like commonly used CNNs, liNets are locally-connected, forming receptive fields. Unlike CNNs, liNets are ideal for irregular photoreceptor distributions like those found in foveated biological retinas. Relative to fully-connected deep neural networks, liNets accommodate a dramatically greater number of retinal photoreceptors for significantly enhanced visual acuity, while avoiding prohibitive memory requirements. We demonstrate that our new networks can serve effectively in the biomimetic active vision system embodied in a simulated human that learns active visuomotor control and active appearance-based recognition.