Tongze He
Paper download is intended for registered attendees only, and is
subjected to the IEEE Copyright Policy. Any other use is strongly forbidden.
Papers from this author
Enhanced User Interest and Expertise Modeling for Expert Recommendation
Tongze He, Caili Guo, Yunfei Chu
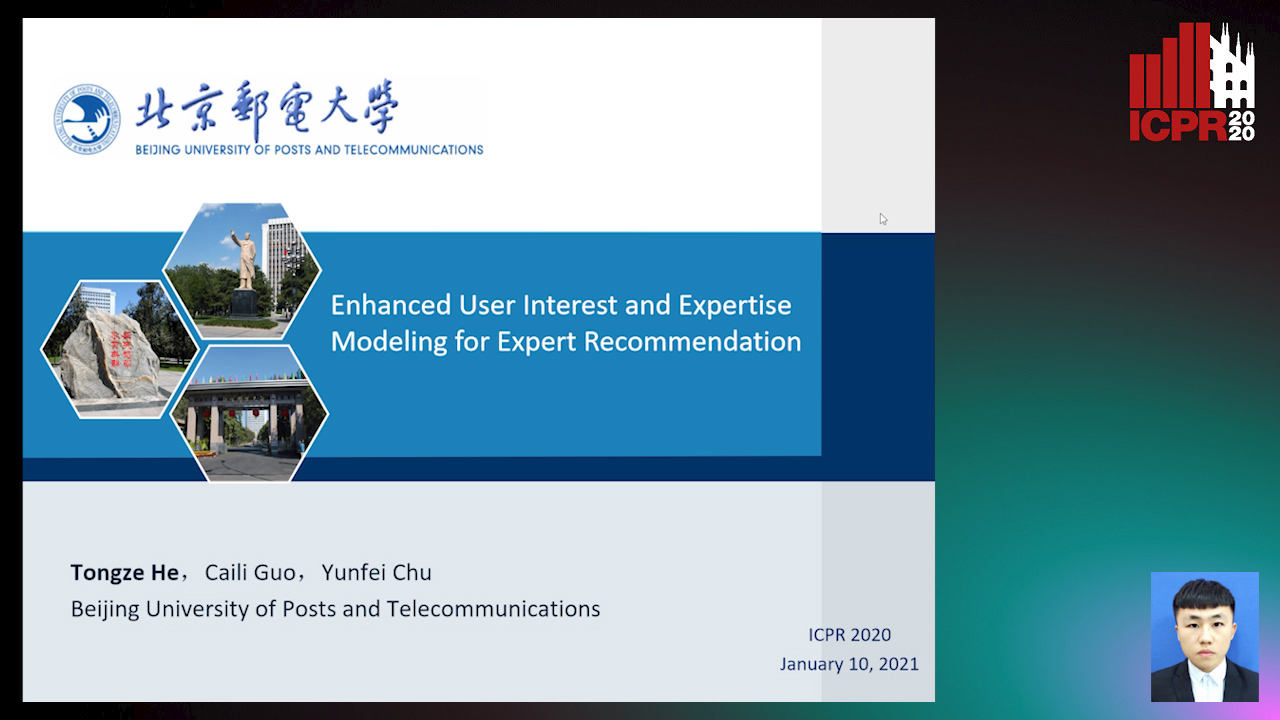
Auto-TLDR; A Unified Framework for Expert Recommendation in Community Question Answering
Abstract Slides Poster Similar
The rapid development of Community Question Answering (CQA) satisfies users' request for professional and personal knowledge. In CQA, one key issue is to recommend users with high expertise and willingness to answer the given questions, namely expert recommendation. However, most of existing methods for expert recommendation ignore some key information, such as time information and historical feedback information, degrading the performance. On the one hand, users' interest are changing over time. It is biased if we don't consider the dynamics. On the other hand, feedback information is critical to estimate users' expertise. To solve these problems, we propose a unified framework for expert recommendation to exploit user interest and expertise more precisely. Considering the inconsistency between them, we propose to learn their embeddings separately. We leverage Long Short-Term Memory (LSTM) to model user's short-term interest and combine it with long-term interest. The user expertise is learned by the designed user expertise network, which explicitly models feedback on users' historical behavior. The extensive experiments on a large-scale dataset from a real-world CQA site demonstrate the superior performance of our method than state-of-the-art solutions to the problem.