Dimitrios Thilikos
Paper download is intended for registered attendees only, and is
subjected to the IEEE Copyright Policy. Any other use is strongly forbidden.
Papers from this author
Hcore-Init: Neural Network Initialization Based on Graph Degeneracy
Stratis Limnios, George Dasoulas, Dimitrios Thilikos, Michalis Vazirgiannis
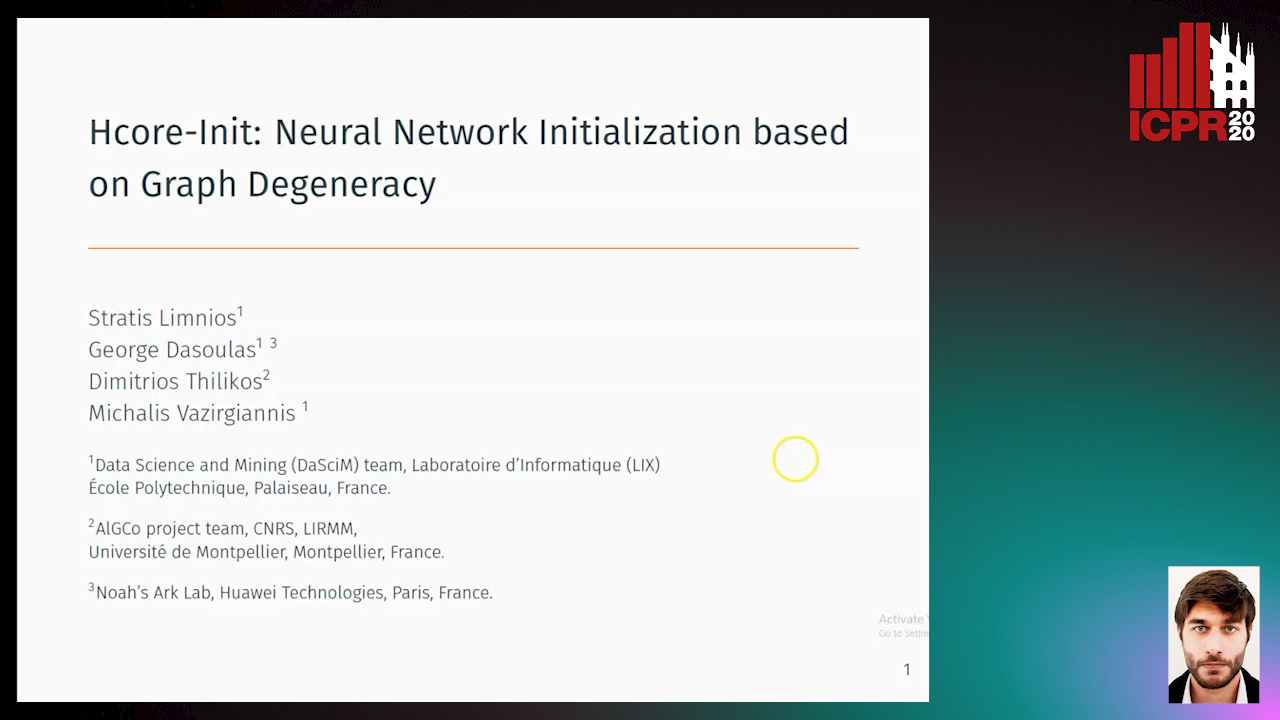
Auto-TLDR; K-hypercore: Graph Mining for Deep Neural Networks
Abstract Slides Poster Similar
Neural networks are the pinnacle of Artificial Intelligence, as in recent years we witnessed many novel architectures, learning and optimization techniques for deep learning. Capitalizing on the fact that neural networks inherently constitute multipartite graphs among neuron layers, we aim to analyze directly their structure to extract meaningful information that can improve the learning process. To our knowledge graph mining techniques for enhancing learning in neural networks have not been thoroughly investigated. In this paper we propose an adapted version of the k-core structure for the complete weighted multipartite graph extracted from a deep learning architecture. As a multipartite graph is a combination of bipartite graphs, that are in turn the incidence graphs of hypergraphs, we design k-hypercore decomposition, the hypergraph analogue of k-core degeneracy. We applied k-hypercore to several neural network architectures, more specifically to convolutional neural networks and multilayer perceptrons for image recognition tasks after a very short pretraining. Then we used the information provided by the hypercore numbers of the neurons to re-initialize the weights of the neural network, thus biasing the gradient optimization scheme. Extensive experiments proved that k-hypercore outperforms the state-of-the-art initialization methods.