Yufan Hou
Paper download is intended for registered attendees only, and is
subjected to the IEEE Copyright Policy. Any other use is strongly forbidden.
Papers from this author
Task-based Focal Loss for Adversarially Robust Meta-Learning
Yufan Hou, Lixin Zou, Weidong Liu
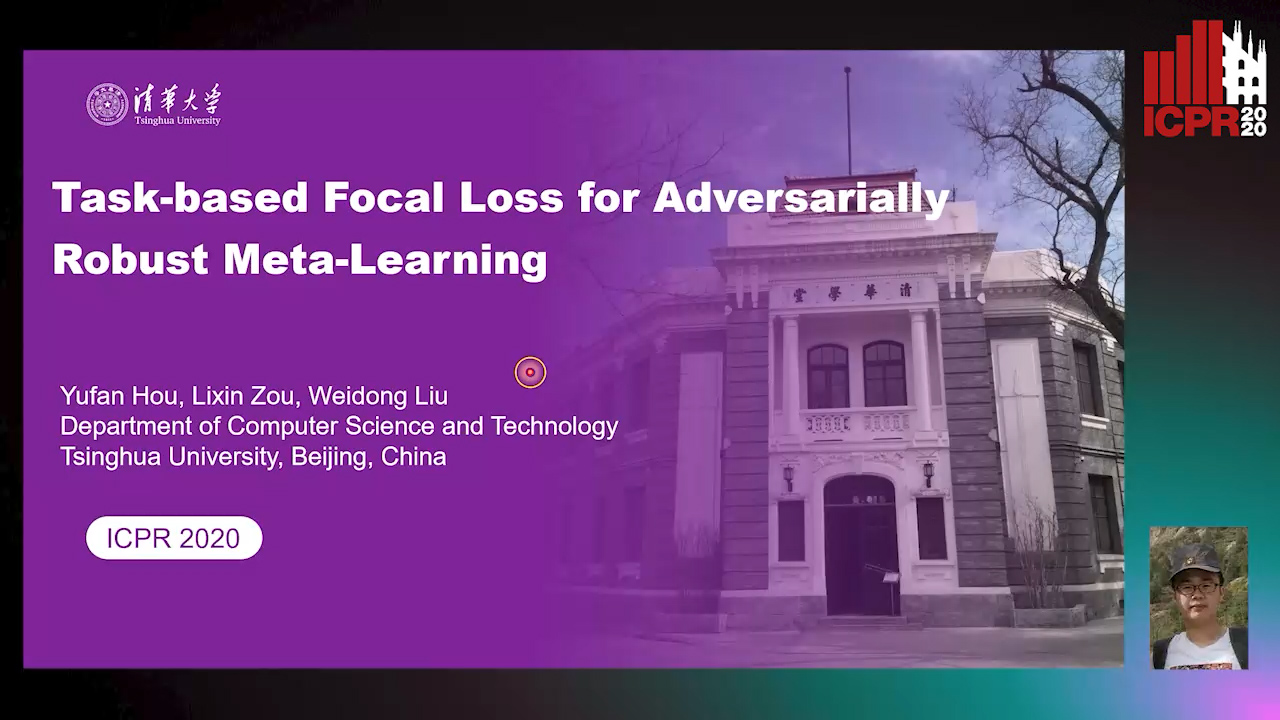
Auto-TLDR; Task-based Adversarial Focal Loss for Few-shot Meta-Learner
Abstract Slides Poster Similar
Adversarial robustness of machine learning has been widely studied in recent years, and a series of effective methods are proposed to resist adversarial attacks. However, less attention is paid to few-shot meta-learners which are much more vulnerable due to the lack of training samples. In this paper, we propose Task-based Adversarial Focal Loss (TAFL) to handle this tough challenge on a typical meta-learner called MAML. More concretely, we regard few-shot classification tasks as normal samples in learning models and apply focal loss mechanism on them. Our proposed method focuses more on adversarially fragile tasks, leading to improvement on overall model robustness. Results of extensive experiments on several benchmarks demonstrate that TAFL can effectively promote the performance of the meta-learner on adversarial examples with elaborately designed perturbations.