Young-Ju Choi
Paper download is intended for registered attendees only, and is
subjected to the IEEE Copyright Policy. Any other use is strongly forbidden.
Papers from this author
Wavelet Attention Embedding Networks for Video Super-Resolution
Young-Ju Choi, Young-Woon Lee, Byung-Gyu Kim
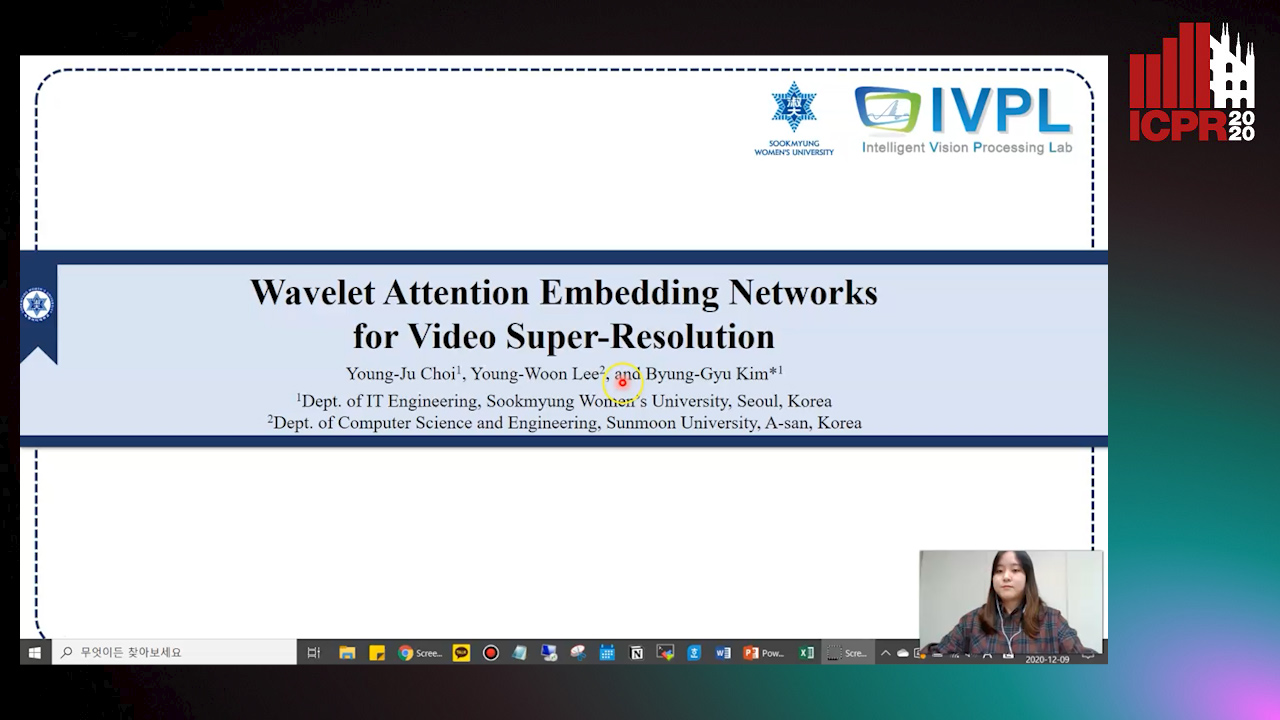
Auto-TLDR; Wavelet Attention Embedding Network for Video Super-Resolution
Abstract Slides Poster Similar
Recently, Video super-resolution (VSR) has become more crucial as the resolution of display has been grown. The majority of deep learning-based VSR methods combine the convolutional neural networks (CNN) with motion compensation or alignment module to estimate high-resolution (HR) frame from low-resolution (LR) frames. However, most of previous methods deal with the spatial features equally and may result in the misaligned temporal features by pixel-based motion compensation and alignment module. It can lead to the damaging effect on the accuracy of the estimated HR feature. In this paper, we propose a wavelet attention embedding network (WAEN), including wavelet embedding network (WENet) and attention embedding network (AENet), to fully exploit the spatio-temporal informative features. The WENet is operated as a spatial feature extractor of individual low and high-frequency information based on 2-D Haar discrete wavelet transform. The meaningful temporal feature is extracted in the AENet through utilizing the weighted attention map between frames. Experimental results demonstrate that the proposed method achieves superior performance compared with state-of-the-art methods.