Victor Finomore
Paper download is intended for registered attendees only, and is
subjected to the IEEE Copyright Policy. Any other use is strongly forbidden.
Papers from this author
XGBoost to Interpret the Opioid Patients’ StateBased on Cognitive and Physiological Measures
Arash Shokouhmand, Omid Dehzangi, Jad Ramadan, Victor Finomore, Nasser M. Nasarabadi, Ali Rezai
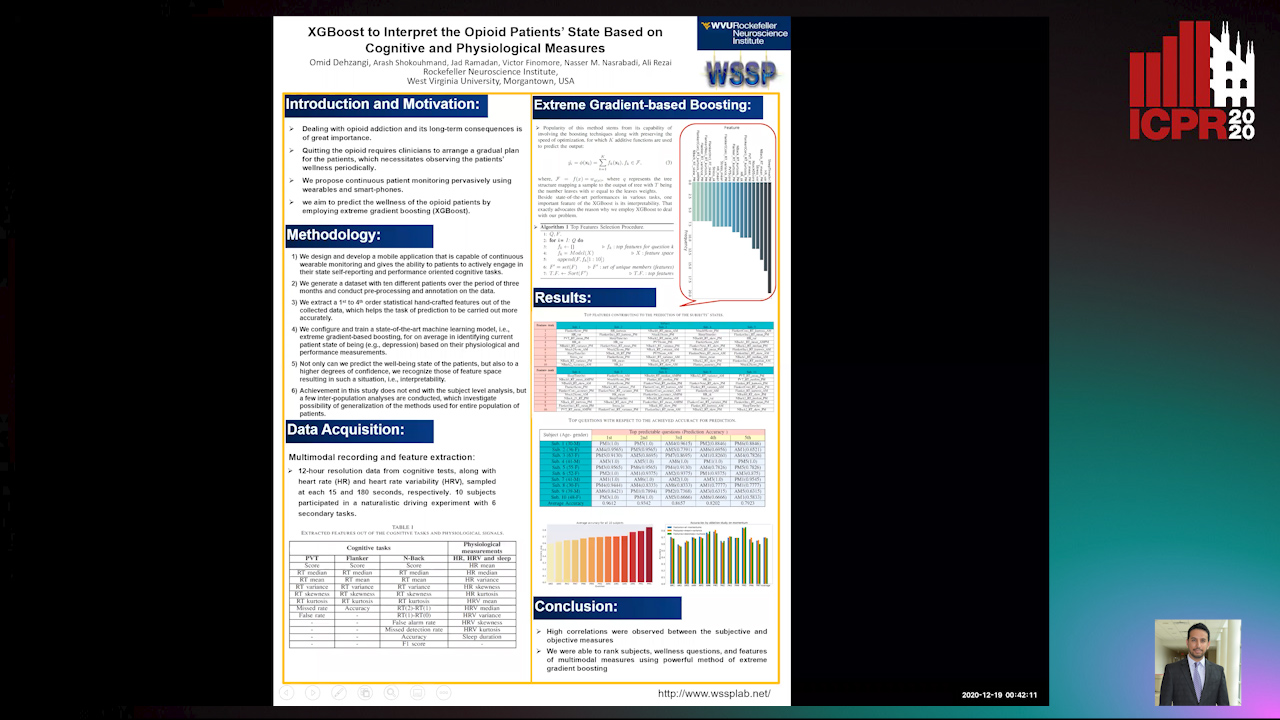
Auto-TLDR; Predicting the Wellness of Opioid Addictions Using Multi-modal Sensor Data
Dealing with opioid addiction and its long-term consequences is of great importance, as the addiction to opioids is emerged gradually, and established strongly in a given patient's body. Based on recent research, quitting the opioid requires clinicians to arrange a gradual plan for the patients who deal with the difficulties of overcoming addiction. This, in turn, necessitates observing the patients' wellness periodically, which is conventionally made by setting clinical appointments. However, this approach of dealing runs the risk of relapse for patients, as there would not be any monitoring between the clinical sessions. Thus, we need to increase the number of clinical appointments for opioid patients, which is not feasible due to the high financial costs, and the patients not having enough forbearance. Nevertheless, with the advent of wearable sensors continuous patient monitoring becomes possible. However, the data collected through the sensors is pervasively noisy, where using sensors with different sampling frequency challenges the data processing. In this work, we handle this problem by using 12-hour resolution data from cognitive tests, along with heart rate (HR) and heart rate variability (HRV), sampled at each 15 and 180 seconds, respectively. The proposed recipe enables us to interpret the multi-modal sensor data as a feature space, where we can predict the wellness of the opioid patients by employing extreme gradient boosting (XGBoost), which results in 96.12% average accuracy of prediction as the best achieved performance.