Niluthpol Mithun
Paper download is intended for registered attendees only, and is
subjected to the IEEE Copyright Policy. Any other use is strongly forbidden.
Papers from this author
Webly Supervised Image-Text Embedding with Noisy Tag Refinement
Niluthpol Mithun, Ravdeep Pasricha, Evangelos Papalexakis, Amit Roy-Chowdhury
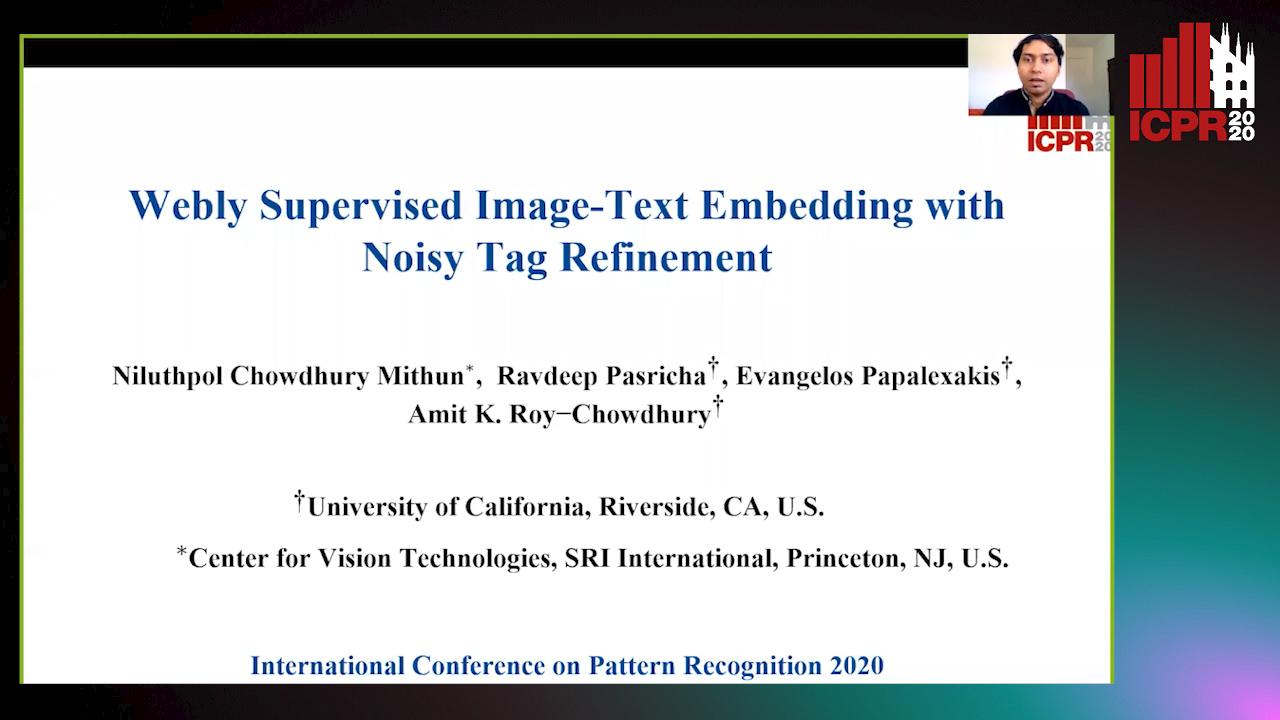
Auto-TLDR; Robust Joint Embedding for Image-Text Retrieval Using Web Images
In this paper, we address the problem of utilizing web images in training robust joint embedding models for the image-text retrieval task. Prior webly supervised approaches directly leverage weakly annotated web images in the joint embedding learning framework. The objective of these approaches would suffer significantly when the ratio of noisy and missing tags associated with the web images is very high. In this regard, we propose a CP decomposition based tensor completion framework to refine the tags of web images by modeling observed ternary inter-relations between the sets of labeled images, tags, and web images as a tensor. To effectively deal with the high ratio of missing entries likely in our case, we incorporate intra-modal correlation as side information in the proposed framework. Our tag refinement approach combined with existing web supervised image-text embedding approaches provide a more principled way for learning the joint embedding models in the presence of significant noise from web data and limited clean labeled data. Experiments on benchmark datasets demonstrate that the proposed approach helps to achieve a significant performance gain in image-text retrieval.