Prachi Kulkarni
Paper download is intended for registered attendees only, and is
subjected to the IEEE Copyright Policy. Any other use is strongly forbidden.
Papers from this author
A Bayesian Deep CNN Framework for Reconstructing K-T-Undersampled Resting-fMRI
Karan Taneja, Prachi Kulkarni, Shabbir Merchant, Suyash Awate
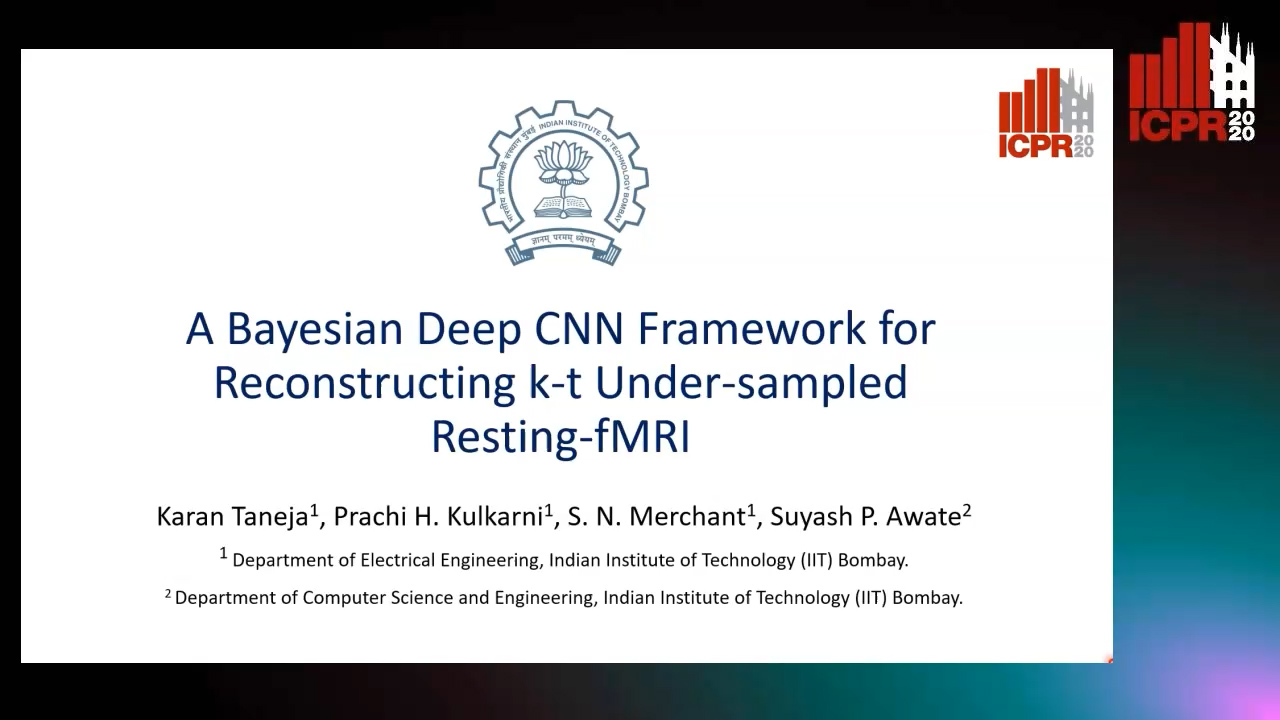
Auto-TLDR; K-t undersampled R-fMRI Reconstruction using Deep Convolutional Neural Networks
Abstract Slides Poster Similar
Undersampled reconstruction in resting functional magnetic resonance imaging (R-fMRI) holds the potential to enable higher spatial resolution in brain R-fMRI without increasing scan duration. We propose a novel framework to reconstruct k-t undersampled R-fMRI relying on a deep convolutional neural network (CNN) framework that leverages the insight that R-fMRI measurements are in k-space (frequency domain) and explicitly models the Fourier transformation from the frequency domain to the spatial domain. The architecture of our CNN framework comprises a multi-stage scheme that jointly learns two multilayer CNN components for (i)~filling in missing k-space data using acquired data in frequency-temporal neighborhoods and (ii)~image quality enhancement in the spatiotemporal domain. We propose four methods within our framework, including a Bayesian CNN that produces uncertainty maps indicating the per-voxel (and per-timepoint) confidence in the blood oxygenation level dependent (BOLD) time-series reconstruction. Results on brain R-fMRI show that our CNN framework improves over the state of the art, quantitatively and qualitatively, in terms of the connectivity maps for three cerebral functional networks.