Subhrajyoti Dasgupta
Paper download is intended for registered attendees only, and is
subjected to the IEEE Copyright Policy. Any other use is strongly forbidden.
Papers from this author
CardioGAN: An Attention-Based Generative Adversarial Network for Generation of Electrocardiograms
Subhrajyoti Dasgupta, Sudip Das, Ujjwal Bhattacharya
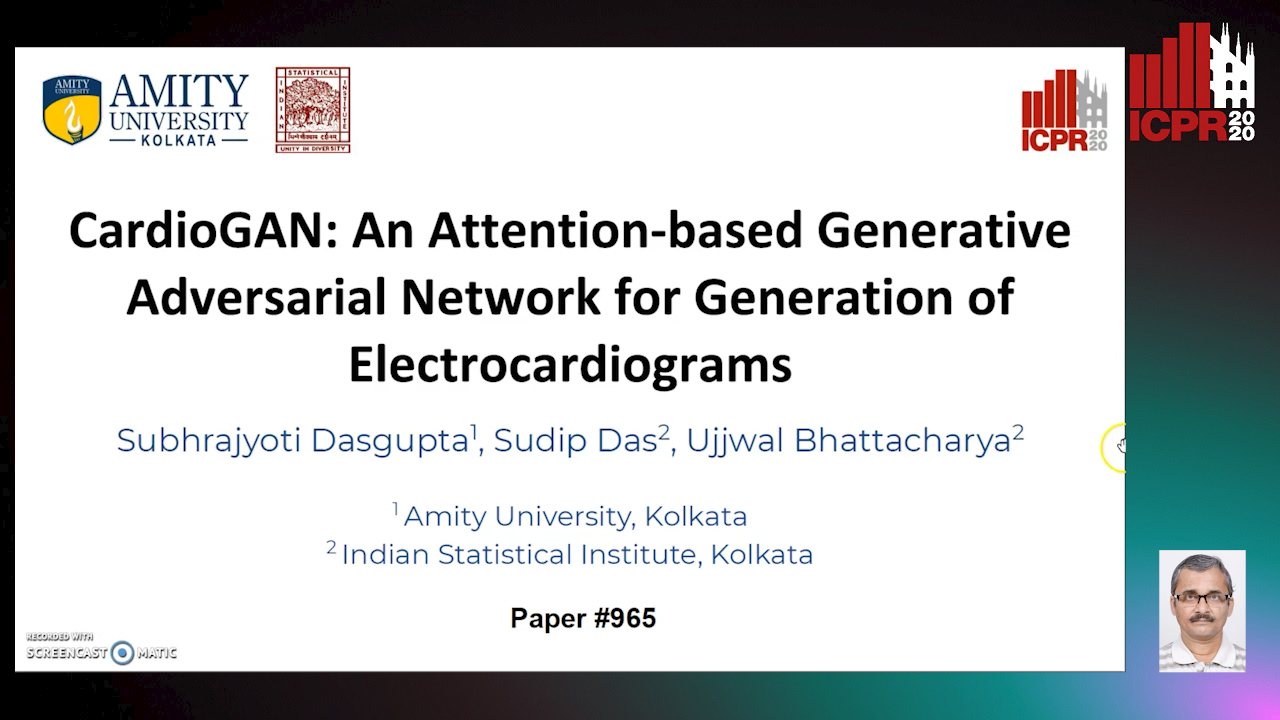
Auto-TLDR; CardioGAN: Generative Adversarial Network for Synthetic Electrocardiogram Signals
Abstract Slides Poster Similar
Electrocardiogram (ECG) signal is studied to obtain crucial information about the condition of a patient's heart. Machine learning based automated medical diagnostic systems that may help to evaluate the condition of the heart from this signal are required to be trained using large volumes of labelled training samples and the same may increase the chance of compromising with the patients' privacy. To solve this issue, generation of synthetic electrocardiogram signals by learning only from the general distributions of the available real training samples have been attempted in the literature. However, these studies did not pay necessary attention to the specific vital details of these signals, such as the P wave, the QRS complex, and the T wave. This shortcoming often results in the generation of unrealistic synthetic signals, such as a signal which does not contain one or more of the above components. In the present study, a novel deep generative architecture, termed as CardioGAN, based on generative adversarial network and powered by the effective attention mechanism has been designed which is capable of learning the intricate inter-dependencies among the various parts of real samples leading to the generation of more realistic electrocardiogram signals. Also, it helps in reducing the risk of breaching the privacy of patients. Extensive experimentation performed by us establishes that the proposed method achieves a better performance in generating synthetic electrocardiogram signals in comparison to the existing methods. The source code will be made available on github.