Mohsen Kheirandishfard
Paper download is intended for registered attendees only, and is
subjected to the IEEE Copyright Policy. Any other use is strongly forbidden.
Papers from this author
Class Conditional Alignment for Partial Domain Adaptation
Mohsen Kheirandishfard, Fariba Zohrizadeh, Farhad Kamangar
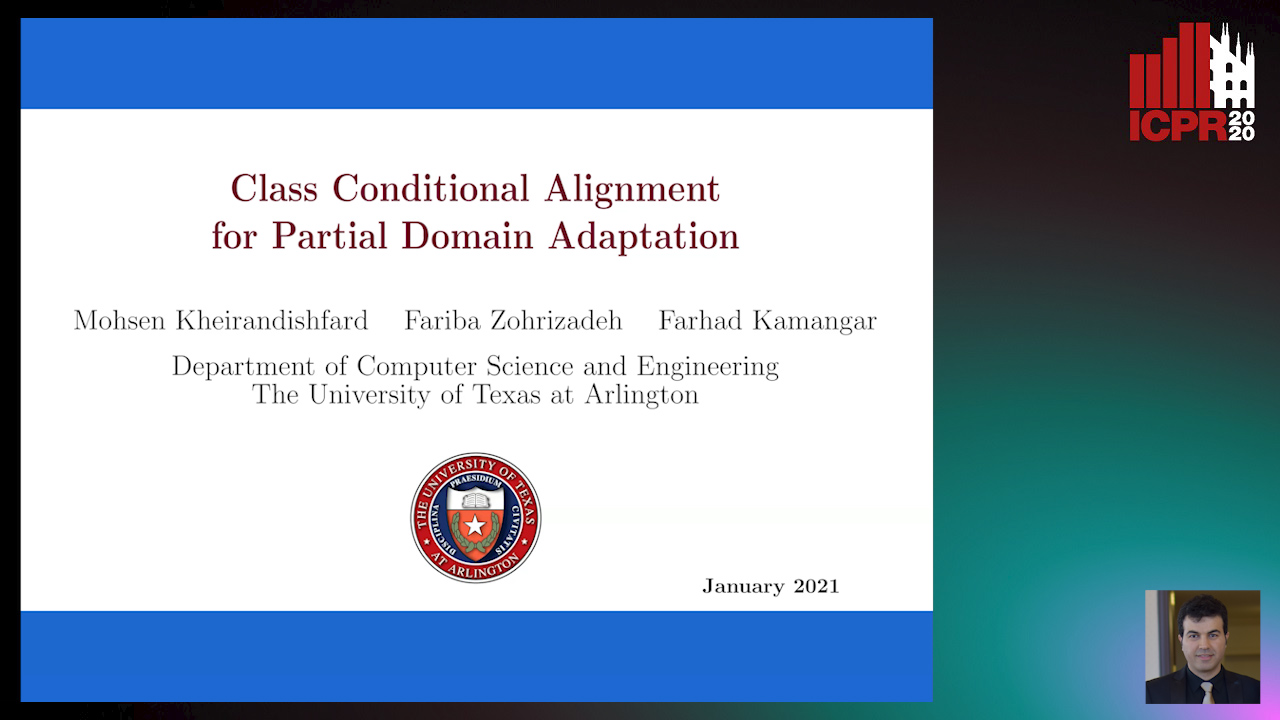
Auto-TLDR; Multi-class Adversarial Adaptation for Partial Domain Adaptation
Abstract Slides Poster Similar
Adversarial adaptation models have demonstrated significant progress towards transferring knowledge from a labeled source dataset to an unlabeled target dataset. Partial domain adaptation (PDA) investigates the scenarios in which the source domain is large and diverse, and the target label space is a subset of the source label space. The main purpose of PDA is to identify the shared classes between the domains and promote learning transferable knowledge from these classes. In this paper, we propose a multi-class adversarial architecture for PDA. The proposed approach jointly aligns the marginal and class-conditional distributions in the shared label space by minimaxing a novel multi-class adversarial loss function. Furthermore, we incorporate effective regularization terms to encourage selecting the most relevant subset of source domain classes. In the absence of target labels, the proposed approach is able to effectively learn domain-invariant feature representations, which in turn can enhance the classification performance in the target domain. Comprehensive experiments on three benchmark datasets Office-$31$, Office-Home, and Caltech-Office corroborate the effectiveness of the proposed approach in addressing different partial transfer learning tasks.