Yingnan Ma
Paper download is intended for registered attendees only, and is
subjected to the IEEE Copyright Policy. Any other use is strongly forbidden.
Papers from this author
Edge-Guided CNN for Denoising Images from Portable Ultrasound Devices
Yingnan Ma, Fei Yang, Anup Basu
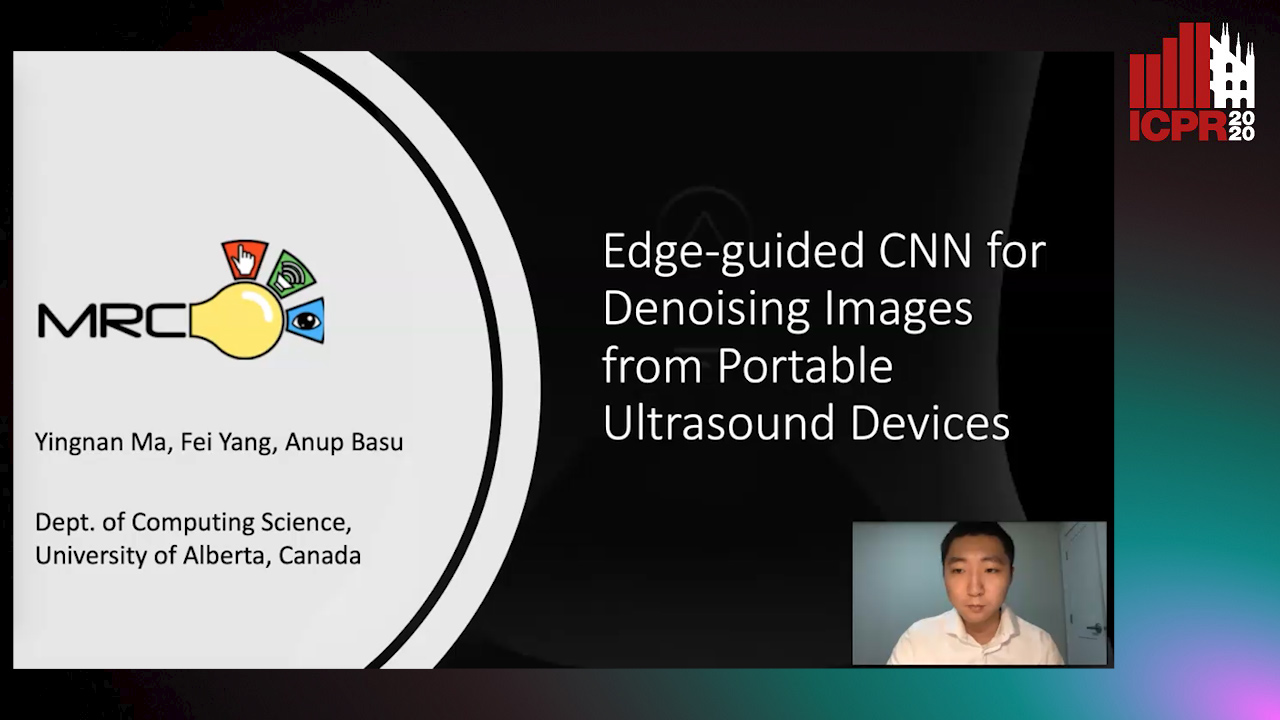
Auto-TLDR; Edge-Guided Convolutional Neural Network for Portable Ultrasound Images
Abstract Slides Poster Similar
Ultrasound is a non-invasive tool that is useful for medical diagnosis and treatment. To reduce long wait times and add convenience to patients, portable ultrasound scanning devices are becoming increasingly popular. These devices can be held in one palm, and are compatible with modern cell phones. However, the quality of ultrasound images captured from the portable scanners is relatively poor compared to standard ultrasound scanning systems in hospitals. To improve the quality of the ultrasound images obtained from portable ultrasound devices, we propose a new neural network architecture called Edge-Guided Convolutional Neural Network (EGCNN), which can preserve significant edge information in ultrasound images when removing noise. We also study and compare the effectiveness of classical filtering approaches in removing speckle noise in these images. Experimental results show that after applying the proposed EGCNN, various organs can be better recognized from ultrasound images. This approach is expected to lead to better accuracy in diagnostics in the future.