Kay Bierzynski
Paper download is intended for registered attendees only, and is
subjected to the IEEE Copyright Policy. Any other use is strongly forbidden.
Papers from this author
Air-Writing with Sparse Network of Radars Using Spatio-Temporal Learning
Muhammad Arsalan, Avik Santra, Kay Bierzynski, Vadim Issakov
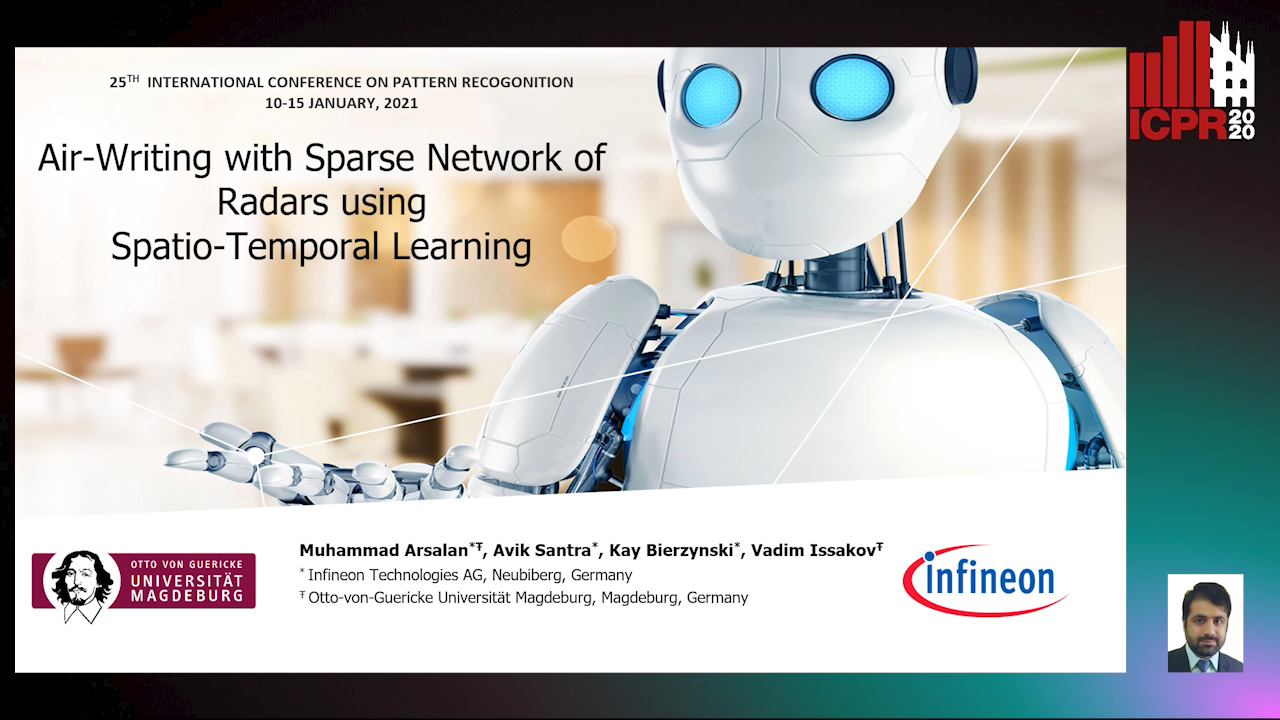
Auto-TLDR; An Air-writing System for Sparse Radars using Deep Convolutional Neural Networks
Abstract Slides Poster Similar
Hand gesture and motion sensing offer an intuitive and natural form of human-machine interface. Air-writing systems allow users to draw alpha-numerical or linguistic characters in the virtual board in air through hand gestures. Traditionally, radar-based air-writing systems have been based on a network of radars, at least three, to localize the hand target through trilateration algorithm followed by tracking to extract the drawn trajectory, which is then followed by recognition of the drawn character by either Long-Short Term Memory (LSTM) utilizing the sensed trajectory or Deep Convolutional Neural Network (DCNN) utilizing a reconstructed 2D image from the trajectory. However, the practical deployments of such systems are limited since the detection of the finger or hand target by all three radars cannot be guaranteed leading to failure of the trilateration algorithm. Further placement of three or more radars for the air-writing solution is neither always physically plausible nor cost-effective. Furthermore, these solutions do not exploit the full potentials of deep neural networks, which are generally capable of learning features implicitly. In this paper, we propose an air-writing system based on a network of sparse radars, i.e. strictly less than three, using 1D DCNN-LSTM-1D transposed DCNN architecture to reconstruct and classify the drawn character utilizing only the range information from each radar. The paper employs real data using one and two 60 GHz milli-meter wave radar sensors to demonstrate the success of the proposed air-writing solution.