Yangbin Chen
Paper download is intended for registered attendees only, and is
subjected to the IEEE Copyright Policy. Any other use is strongly forbidden.
Papers from this author
MetaMix: Improved Meta-Learning with Interpolation-based Consistency Regularization
Yangbin Chen, Yun Ma, Tom Ko, Jianping Wang, Qing Li
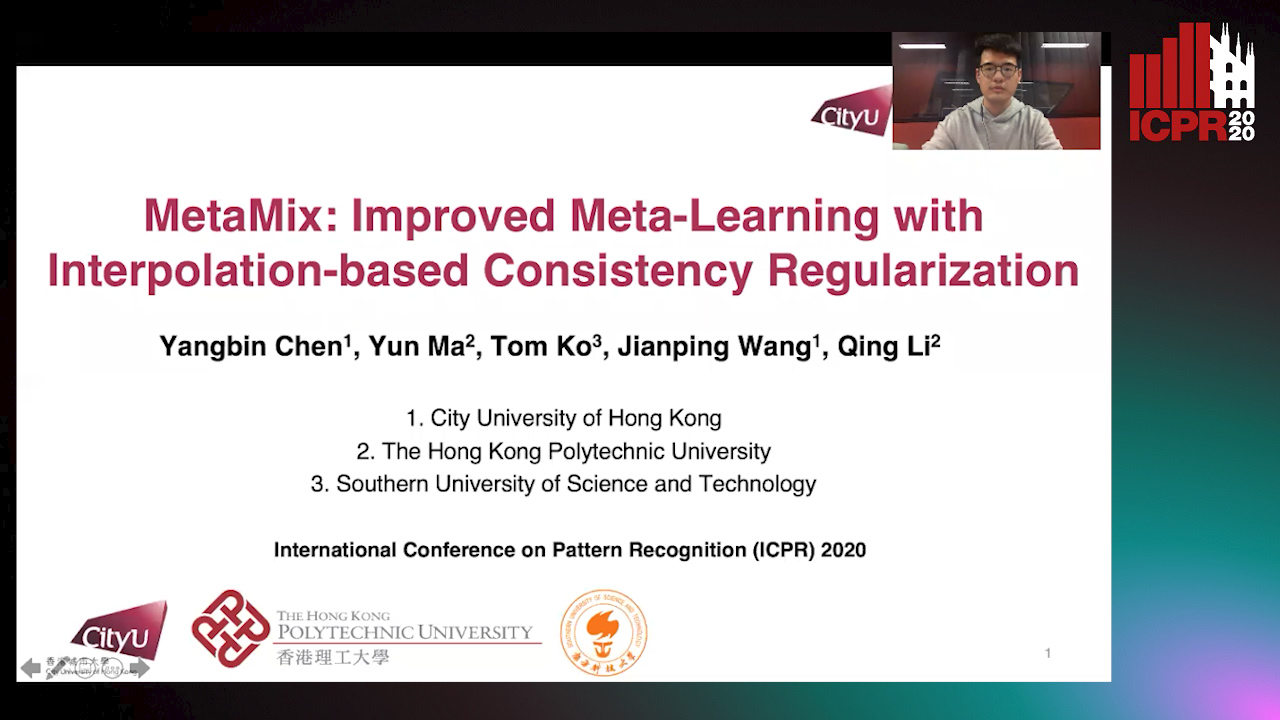
Auto-TLDR; MetaMix: A Meta-Agnostic Meta-Learning Algorithm for Few-Shot Classification
Abstract Slides Poster Similar
Model-Agnostic Meta-Learning (MAML) and its variants are popular few-shot classification methods. They train an initializer across a variety of sampled learning tasks (also known as episodes) such that the initialized model can adapt quickly to new tasks. However, within each episode, current MAML-based algorithms have limitations in forming generalizable decision boundaries using only a few training examples. In this paper, we propose an approach called MetaMix. It generates virtual examples within each episode to regularize the backbone models. MetaMix can be applied in any of the MAML-based algorithms and learn the decision boundaries which are more generalizable to new tasks. Experiments on the mini-ImageNet, CUB, and FC100 datasets show that MetaMix improves the performance of MAML-based algorithms and achieves the state-of-the-art result when applied in Meta-Transfer Learning.