Linlin Zong
Paper download is intended for registered attendees only, and is
subjected to the IEEE Copyright Policy. Any other use is strongly forbidden.
Papers from this author
Constrained Spectral Clustering Network with Self-Training
Xinyue Liu, Shichong Yang, Linlin Zong
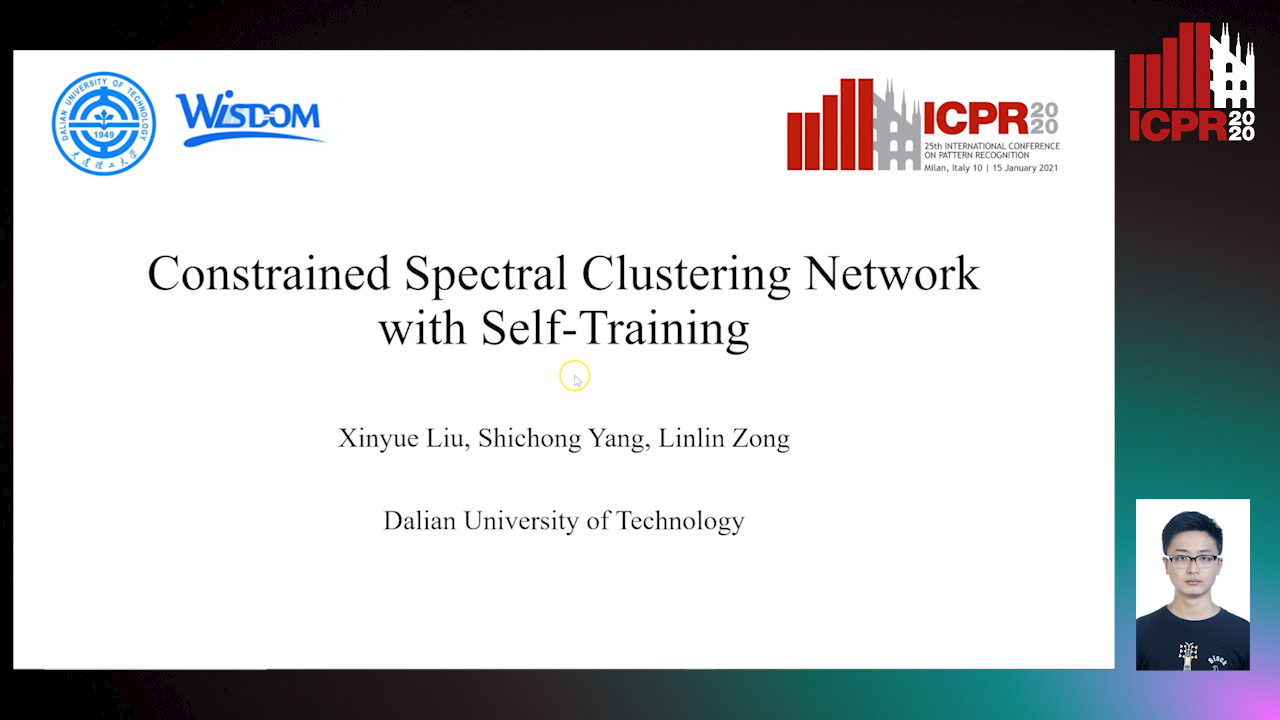
Auto-TLDR; Constrained Spectral Clustering Network: A Constrained Deep spectral clustering network
Abstract Slides Poster Similar
Deep spectral clustering networks have shown their superiorities due to the integration of feature learning and cluster assignment, and the ability to deal with non-convex clusters. Nevertheless, deep spectral clustering is still an ill-posed problem. Specifically, the affinity learned by the most remarkable SpectralNet is not guaranteed to be consistent with local invariance and thus hurts the final clustering performance. In this paper, we propose a novel framework of Constrained Spectral Clustering Network (CSCN) by incorporating pairwise constraints and clustering oriented fine-tuning to deal with the ill-posedness. To the best of our knowledge, this is the first constrained deep spectral clustering method. Another advantage of CSCN over existing constrained deep clustering networks is that it propagates pairwise constraints throughout the entire dataset. In addition, we design a clustering oriented loss by self-training to simultaneously finetune feature representations and perform cluster assignments, which further improve the quality of clustering. Extensive experiments on benchmark datasets demonstrate that our approach outperforms the state-of-the-art clustering methods.