Junjie Wang
Paper download is intended for registered attendees only, and is
subjected to the IEEE Copyright Policy. Any other use is strongly forbidden.
Papers from this author
Heterogeneous Graph-Based Knowledge Transfer for Generalized Zero-Shot Learning
Junjie Wang, Xiangfeng Wang, Bo Jin, Junchi Yan, Wenjie Zhang, Hongyuan Zha
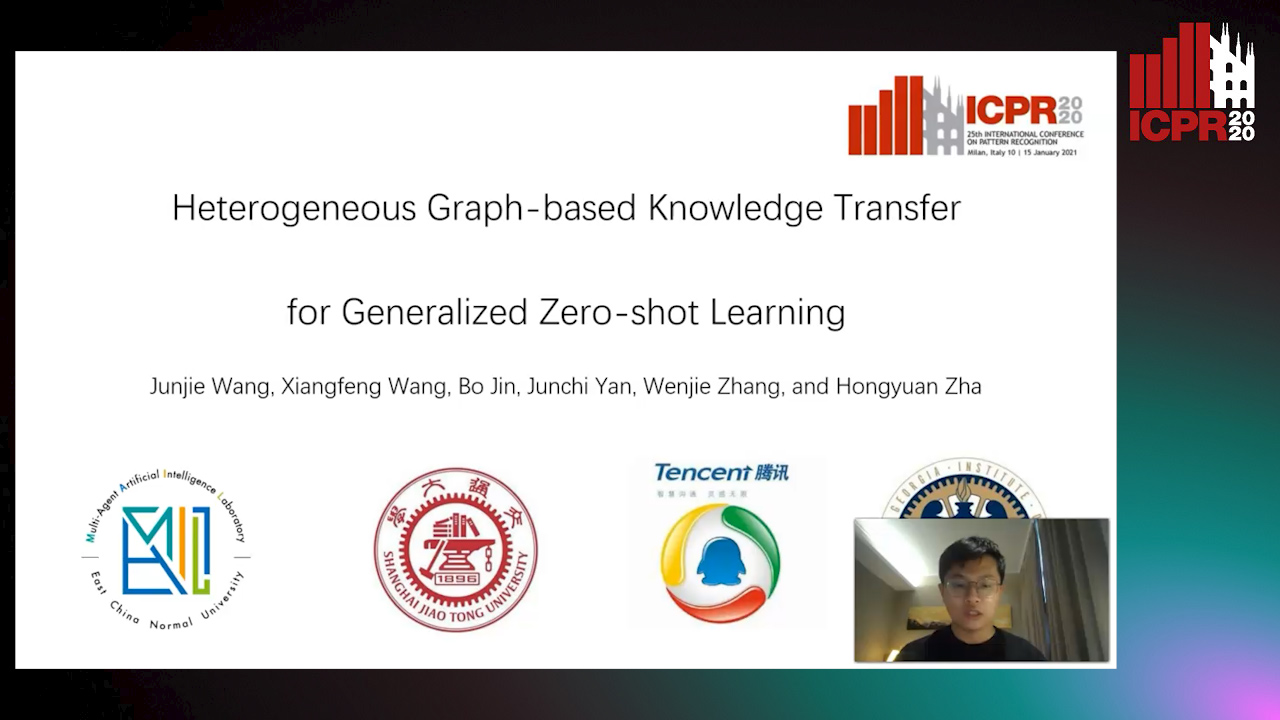
Auto-TLDR; Heterogeneous Graph-based Knowledge Transfer for Generalized Zero-Shot Learning
Abstract Slides Poster Similar
Generalized zero-shot learning (GZSL) tackles the problem of learning to classify instances involving both seen classes and unseen ones. The key issue is how to effectively transfer the model learned from seen classes to unseen classes. Existing works in GZSL usually assume that some prior information about unseen classes are available. However, such an assumption is unrealistic when new unseen classes appear dynamically. To this end, we propose a novel heterogeneous graph-based knowledge transfer method (HGKT) for GZSL, agnostic to unseen classes and instances, by leveraging graph neural network. Specifically, a structured heterogeneous graph is constructed with high-level representative nodes for seen classes, which are chosen through Wasserstein barycenter in order to simultaneously capture inter-class and intra-class relationship. The aggregation and embedding functions can be learned throughgraph neural network, which can be used to compute the embeddings of unseen classes by transferring the knowledge from their neighbors. Extensive experiments on public benchmark datasets show that our method achieves state-of-the-art results.