Yongsheng Bai
Paper download is intended for registered attendees only, and is
subjected to the IEEE Copyright Policy. Any other use is strongly forbidden.
Papers from this author
End-To-End Deep Learning Methods for Automated Damage Detection in Extreme Events at Various Scales
Yongsheng Bai, Alper Yilmaz, Halil Sezen
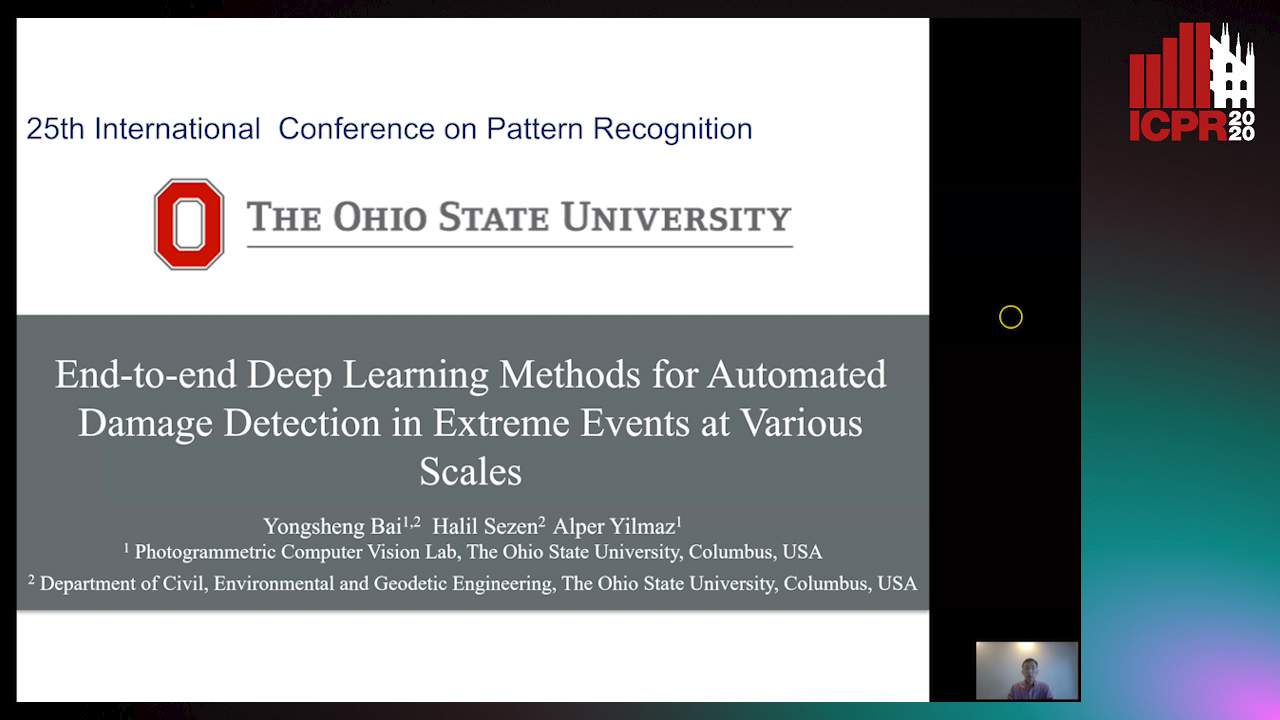
Auto-TLDR; Robust Mask R-CNN for Crack Detection in Extreme Events
Abstract Slides Poster Similar
Robust Mask R-CNN (Mask Regional Convolutional Neural Network) methods are proposed and tested for automatic detection of cracks on structures or their components that may be damaged during extreme events, such as earth-quakes. We curated a new dataset with 2,021 labeled images for training and validation and aimed to find end-to-end deep neural networks for crack detection in the field. With data augmentation and parameters fine-tuning, Path Aggregation Network (PANet) with spatial attention mechanisms and High-resolution Network (HRNet) are introduced into Mask R-CNNs. The tests on three public datasets with low- or high-resolution images demonstrate that the proposed methods can achieve a big improvement over alternative networks, so the proposed method may be sufficient for crack detection for a variety of scales in real applications.