Hongliu Cao
Paper download is intended for registered attendees only, and is
subjected to the IEEE Copyright Policy. Any other use is strongly forbidden.
Papers from this author
A Novel Random Forest Dissimilarity Measure for Multi-View Learning
Hongliu Cao, Simon Bernard, Robert Sabourin, Laurent Heutte
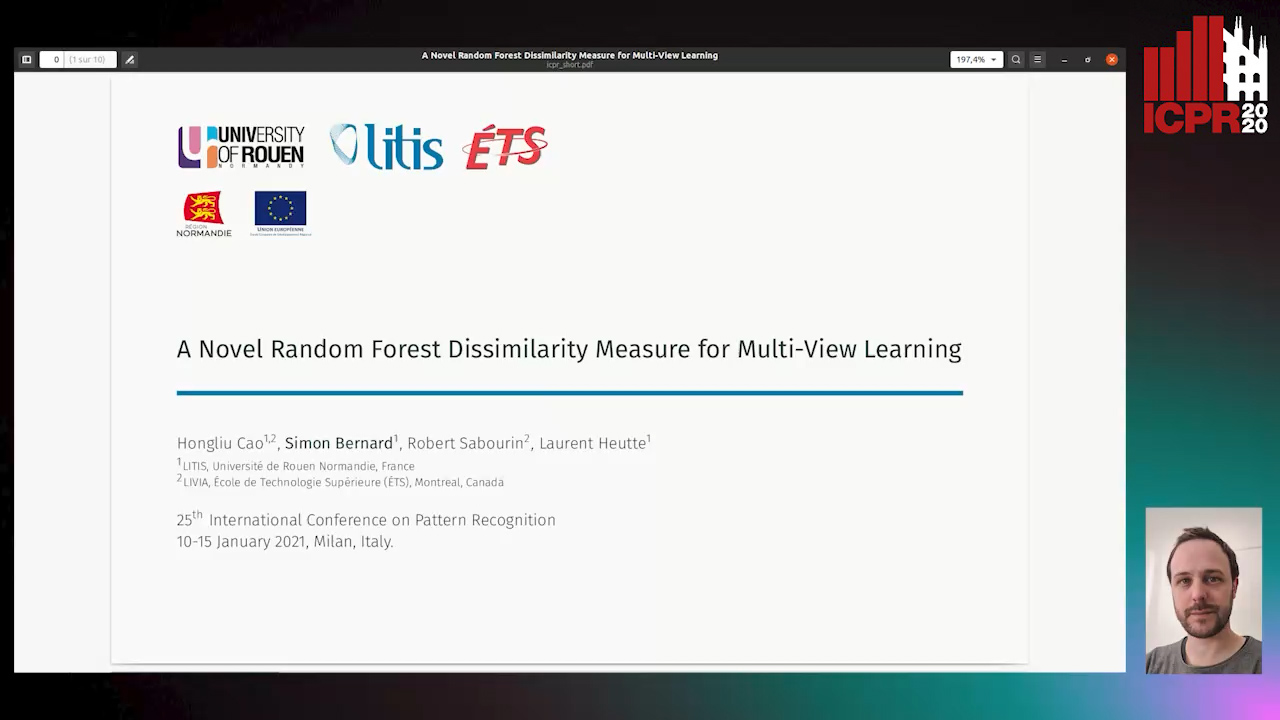
Auto-TLDR; Multi-view Learning with Random Forest Relation Measure and Instance Hardness
Abstract Slides Poster Similar
Multi-view learning is a learning task in which data is described by several concurrent representations. Its main challenge is most often to exploit the complementarities between these representations to help solve a classification/regression task. This is a challenge that can be met nowadays if there is a large amount of data available for learning. However, this is not necessarily true for all real-world problems, where data are sometimes scarce (e.g. problems related to the medical environment). In these situations, an effective strategy is to use intermediate representations based on the dissimilarities between instances. This work presents new ways of constructing these dissimilarity representations, learning them from data with Random Forest classifiers. More precisely, two methods are proposed, which modify the Random Forest proximity measure, to adapt it to the context of High Dimension Low Sample Size (HDLSS) multi-view classification problems. The second method, based on an Instance Hardness measurement, is significantly more accurate than other state-of-the-art measurements including the original RF Proximity measurement and the Large Margin Nearest Neighbor (LMNN) metric learning measurement.