Antonella Mensi
Paper download is intended for registered attendees only, and is
subjected to the IEEE Copyright Policy. Any other use is strongly forbidden.
Papers from this author
Proximity Isolation Forests
Antonella Mensi, Manuele Bicego, David Tax
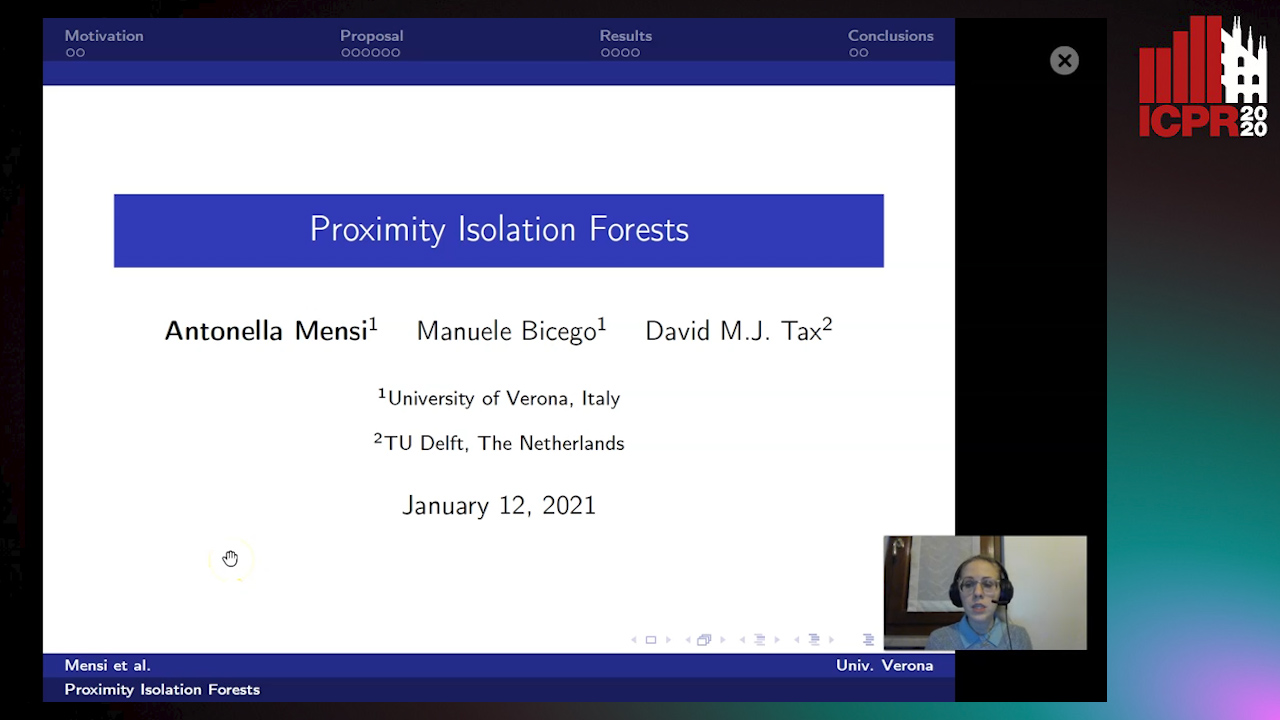
Auto-TLDR; Proximity Isolation Forests for Non-vectorial Data
Abstract Slides Poster Similar
Isolation Forests are a very successful approach for solving outlier detection tasks. Isolation Forests are based on classical Random Forest classifiers that require feature vectors as input. There are many situations where vectorial data is not readily available, for instance when dealing with input sequences or strings. In these situations, one can extract higher level characteristics from the input, which is typically hard and often loses valuable information. An alternative is to define a proximity between the input objects, which can be more intuitive. In this paper we propose the Proximity Isolation Forests that extend the Isolation Forests to non-vectorial data. The introduced methodology has been thoroughly evaluated on 8 different problems and it achieves very good results also when compared to other techniques.