Masayuki Tanaka
Paper download is intended for registered attendees only, and is
subjected to the IEEE Copyright Policy. Any other use is strongly forbidden.
Papers from this author
Human Segmentation with Dynamic LiDAR Data
Tao Zhong, Wonjik Kim, Masayuki Tanaka, Masatoshi Okutomi
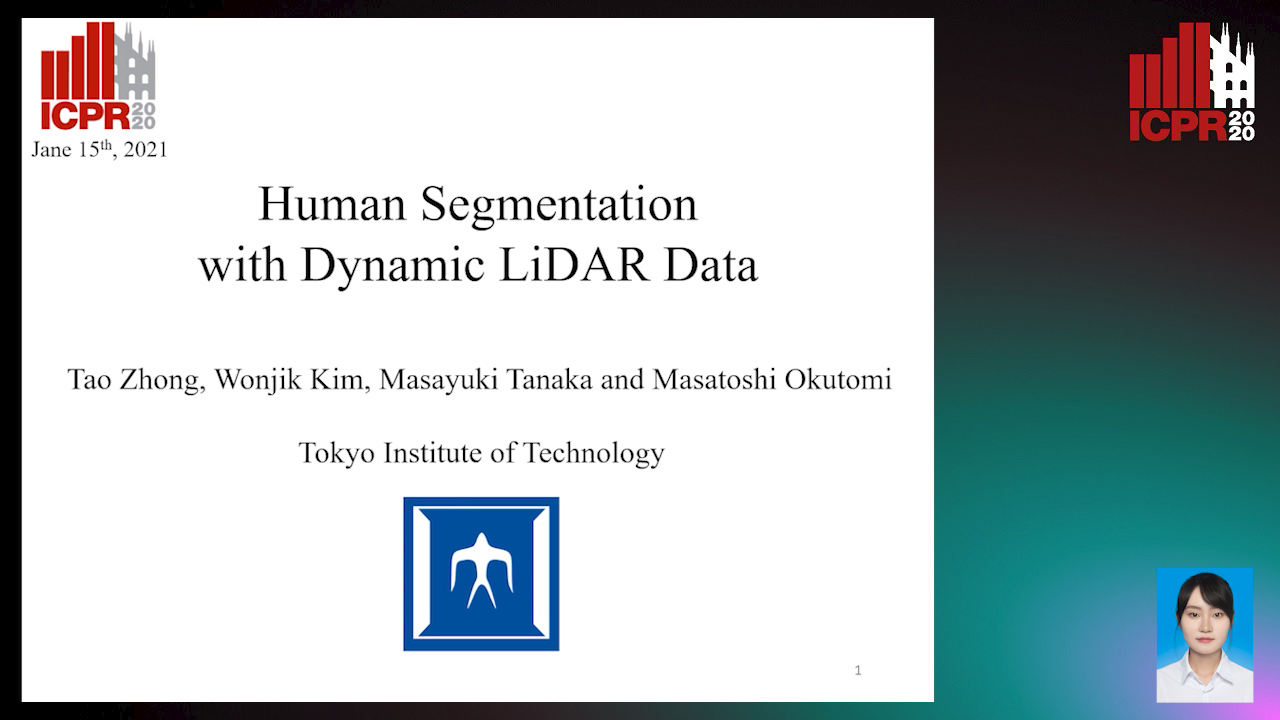
Auto-TLDR; Spatiotemporal Neural Network for Human Segmentation with Dynamic Point Clouds
Consecutive LiDAR scans and depth images compose dynamic 3D sequences, which contain more abundant spatiotemporal information than a single frame. Similar to the development history of image and video perception, dynamic 3D sequence perception starts to come into sight after inspiring research on static 3D data perception. This work proposes a spatiotemporal neural network for human segmentation with the dynamic LiDAR point clouds. It takes a sequence of depth images as input. It has a two-branch structure, i.e., the spatial segmentation branch and the temporal velocity estimation branch. The velocity estimation branch is designed to capture motion cues from the input sequence and then propagates them to the other branch. So that the segmentation branch segments humans according to both spatial and temporal features. These two branches are jointly learned on a generated dynamic point cloud data set for human recognition. Our works fill in the blank of dynamic point cloud perception with the spherical representation of point cloud and achieves high accuracy. The experiments indicate that the introduction of temporal feature benefits the segmentation of dynamic point cloud perception.