Hui Li
Paper download is intended for registered attendees only, and is
subjected to the IEEE Copyright Policy. Any other use is strongly forbidden.
Papers from this author
Subspace Clustering Via Joint Unsupervised Feature Selection
Wenhua Dong, Xiaojun Wu, Hui Li, Zhenhua Feng, Josef Kittler
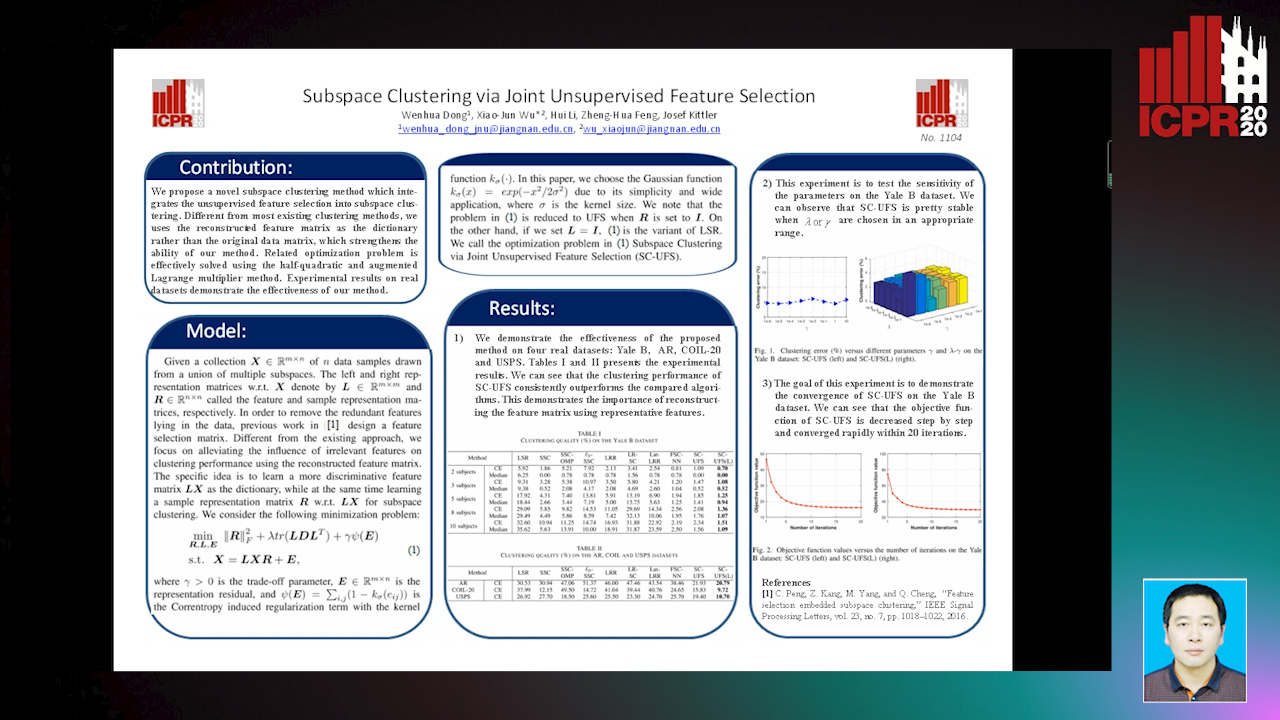
Auto-TLDR; Unsupervised Feature Selection for Subspace Clustering
Any high-dimensional data arising from practical applications usually contains irrelevant features, which may impact on the performance of existing subspace clustering methods. This paper proposes a novel subspace clustering method, which reconstructs the feature matrix by the means of unsupervised feature selection (UFS) to achieve a better dictionary for subspace clustering (SC). Different from most existing clustering methods, the proposed approach uses a reconstructed feature matrix as the dictionary rather than the original data matrix. As the feature matrix reconstructed by representative features is more discriminative and closer to the ground-truth, it results in improved performance. The corresponding non-convex optimization problem is effectively solved using the half-quadratic and augmented Lagrange multiplier methods. Extensive experiments on four real datasets demonstrate the effectiveness of the proposed method.