Zhiwei Wen
Paper download is intended for registered attendees only, and is
subjected to the IEEE Copyright Policy. Any other use is strongly forbidden.
Papers from this author
Group-Wise Feature Orthogonalization and Suppression for GAN Based Facial Attribute Translation
Zhiwei Wen, Haoqian Wu, Weicheng Xie, Linlin Shen
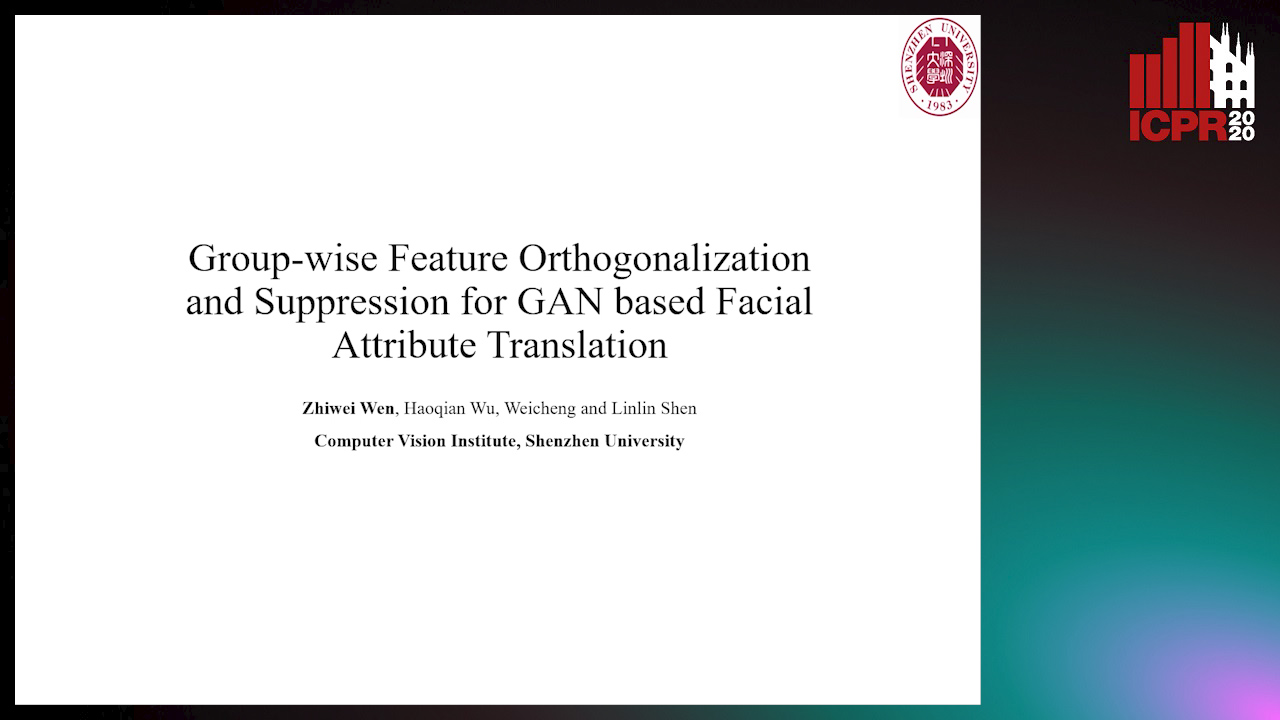
Auto-TLDR; Semantic Disentanglement of Generative Adversarial Network
Abstract Slides Poster Similar
Generative Adversarial Network (GAN) has been widely used for object attribute editing. However, the semantic correlation, resulted from the feature map interaction in the generative network of GAN, may impair the generalization ability of the generative network. In this work, semantic disentanglement is introduced in GAN to reduce the attribute correlation. The feature maps of the generative network are first grouped with an efficient clustering algorithm based on hash encoding, which are used to excavate hidden semantic attributes and calculate the group-wise orthogonality loss for the reduction of attribute entanglement. Meanwhile, the feature maps falling in the intersection regions of different groups are further suppressed to reduce the attribute-wise interaction. Extensive experiments reveal that the proposed GAN generated more genuine objects than the state of the arts. Quantitative results of classification accuracy, inception and FID scores further justify the effectiveness of the proposed GAN.