Fernando Moya Rueda
Paper download is intended for registered attendees only, and is
subjected to the IEEE Copyright Policy. Any other use is strongly forbidden.
Papers from this author
From Human Pose to On-Body Devices for Human-Activity Recognition
Fernando Moya Rueda, Gernot Fink
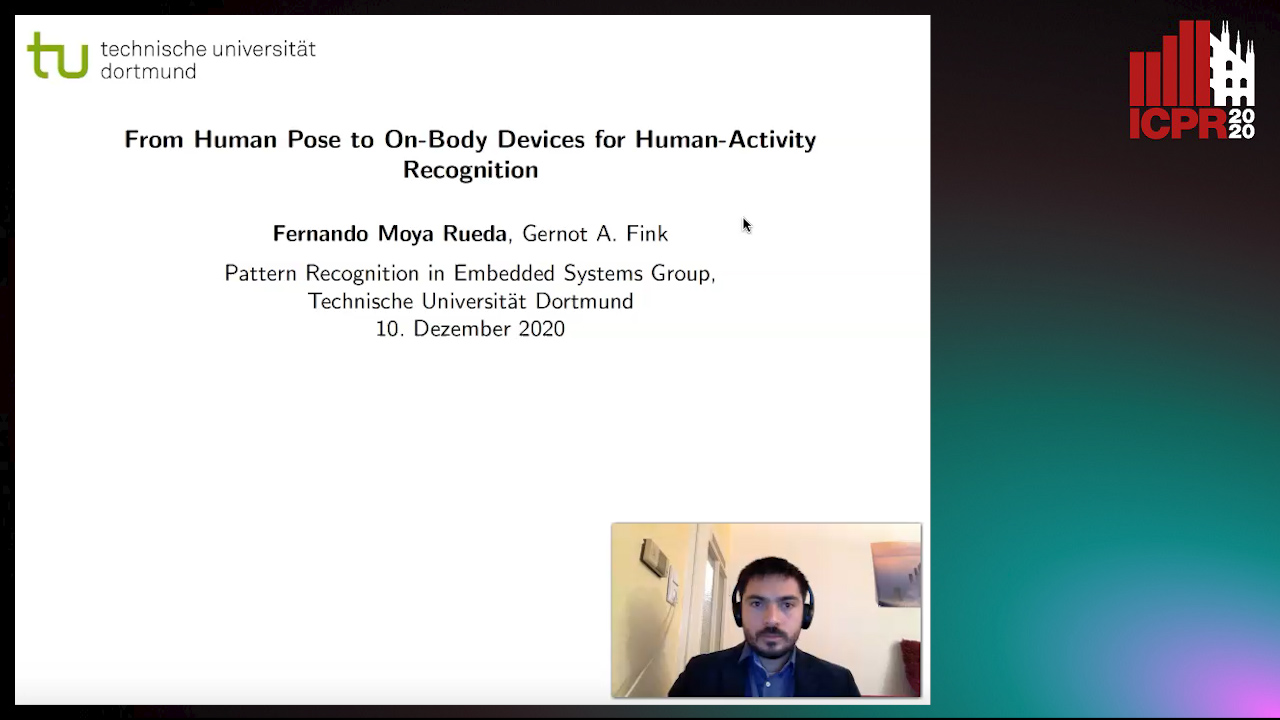
Auto-TLDR; Transfer Learning from Human Pose Estimation for Human Activity Recognition using Inertial Measurements from On-Body Devices
Abstract Slides Poster Similar
Human Activity Recognition (HAR), using inertial measurements from on-body devices, has not seen a great advantage from deep architectures. This is mainly due to the lack of annotated data, diversity of on-body device configurations, the class-unbalance problem, and non-standard human activity definitions. Approaches for improving the performance of such architectures, e.g., transfer learning, are therefore difficult to apply. This paper introduces a method for transfer learning from human-pose estimations as a source for improving HAR using inertial measurements obtained from on-body devices. We propose to fine-tune deep architectures, trained using sequences of human poses from a large dataset and their derivatives, for solving HAR on inertial measurements from on-body devices. Derivatives of human poses will be considered as a sort of synthetic data for HAR. We deploy two different temporal-convolutional architectures as classifiers. An evaluation of the method is carried out on three benchmark datasets improving the classification performance.