Anne Haake
Paper download is intended for registered attendees only, and is
subjected to the IEEE Copyright Policy. Any other use is strongly forbidden.
Papers from this author
Interpretable Structured Learning with Sparse Gated Sequence Encoder for Protein-Protein Interaction Prediction
Kishan K C, Feng Cui, Anne Haake, Rui Li
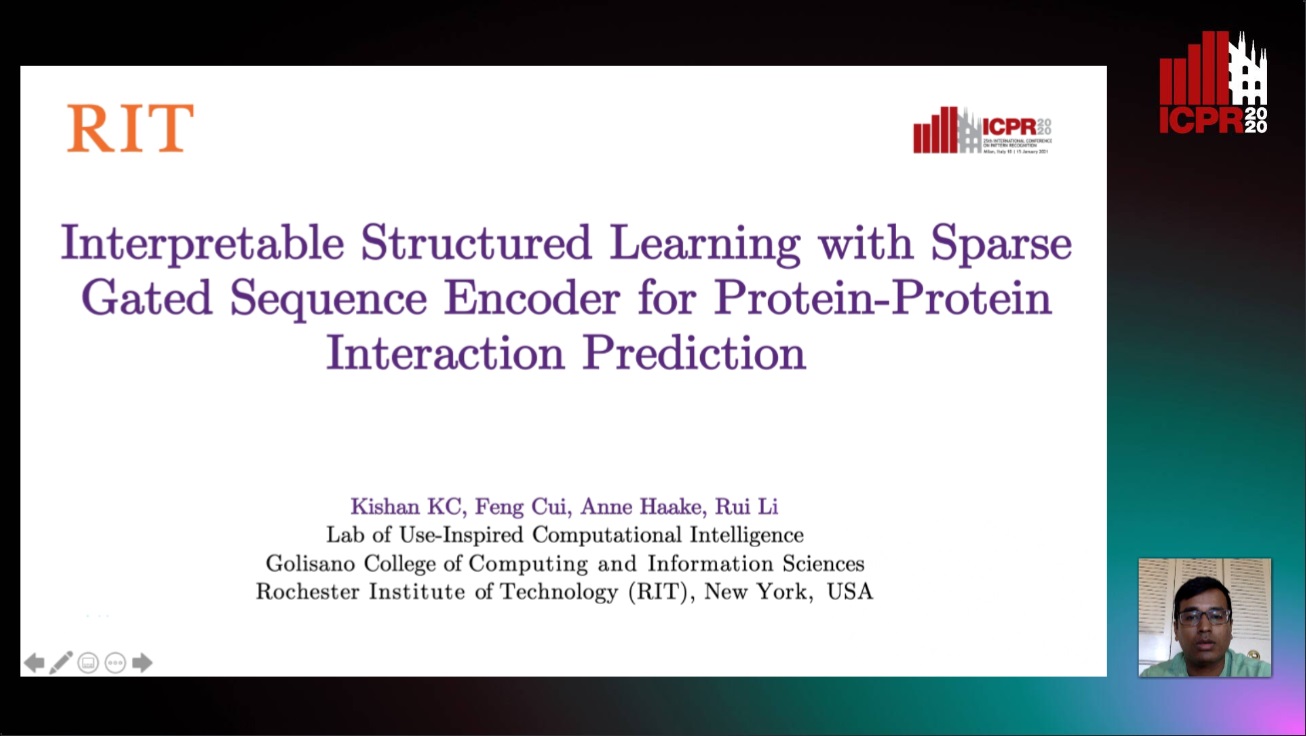
Auto-TLDR; Predicting Protein-Protein Interactions Using Sequence Representations
Abstract Slides Poster Similar
Predicting protein-protein interactions (PPIs) by learning informative representations from amino acid sequences is a challenging yet important problem in biology. Although various deep learning models in Siamese architecture have been proposed to model PPIs from sequences, these methods are computationally expensive for a large number of PPIs due to the pairwise encoding process. Furthermore, these methods are difficult to interpret because of non-intuitive mappings from protein sequences to their sequence representation. To address these challenges, we present a novel deep framework to model and predict PPIs from sequence alone. Our model incorporates a bidirectional gated recurrent unit to learn sequence representations by leveraging contextualized and sequential information from sequences. We further employ a sparse regularization to model long-range dependencies between amino acids and to select important amino acids (protein motifs), thus enhancing interpretability. Besides, the novel design of the encoding process makes our model computationally efficient and scalable to an increasing number of interactions. Experimental results on up-to-date interaction datasets demonstrate that our model achieves superior performance compared to other state-of-the-art methods. Literature-based case studies illustrate the ability of our model to provide biological insights to interpret the predictions.