Jiyuan Liu
Paper download is intended for registered attendees only, and is
subjected to the IEEE Copyright Policy. Any other use is strongly forbidden.
Papers from this author
Image Representation Learning by Transformation Regression
Xifeng Guo, Jiyuan Liu, Sihang Zhou, En Zhu, Shihao Dong
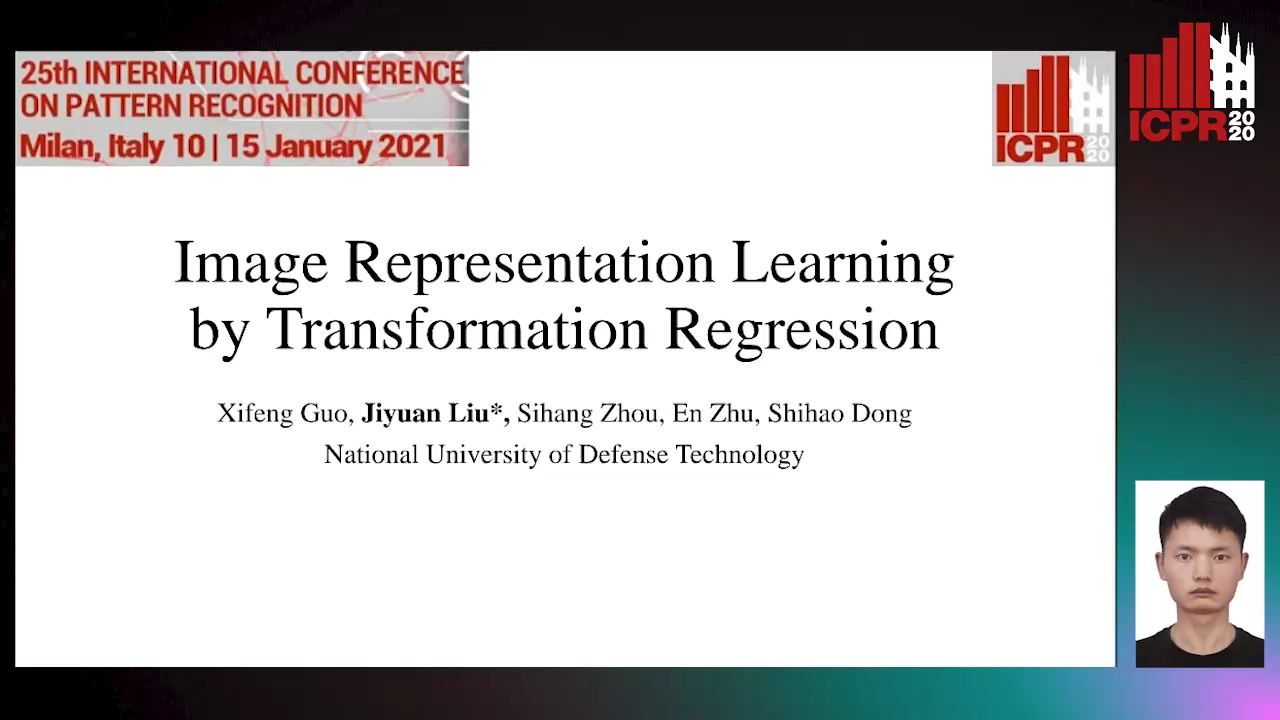
Auto-TLDR; Self-supervised Image Representation Learning using Continuous Parameter Prediction
Abstract Slides Poster Similar
Self-supervised learning is a thriving research direction since it can relieve the burden of human labeling for machine learning by seeking for supervision from data instead of human annotation. Although demonstrating promising performance in various applications, we observe that the existing methods usually model the auxiliary learning tasks as classification tasks with finite discrete labels, leading to insufficient supervisory signals, which in turn restricts the representation quality. In this paper, to solve the above problem and make full use of the supervision from data, we design a regression model to predict the continuous parameters of a group of transformations, i.e., image rotation, translation, and scaling. Surprisingly, this naive modification stimulates tremendous potential from data and the resulting supervisory signal has largely improved the performance of image representation learning. Extensive experiments on four image datasets, including CIFAR10, CIFAR100, STL10, and SVHN, indicate that our proposed algorithm outperforms the state-of-the-art unsupervised learning methods by a large margin in terms of classification accuracy. Crucially, we find that with our proposed training mechanism as an initialization, the performance of the existing state-of-the-art classification deep architectures can be preferably improved.