Hongli Zhou
Paper download is intended for registered attendees only, and is
subjected to the IEEE Copyright Policy. Any other use is strongly forbidden.
Papers from this author
Deep Reinforcement Learning for Autonomous Driving by Transferring Visual Features
Hongli Zhou, Guanwen Zhang, Wei Zhou
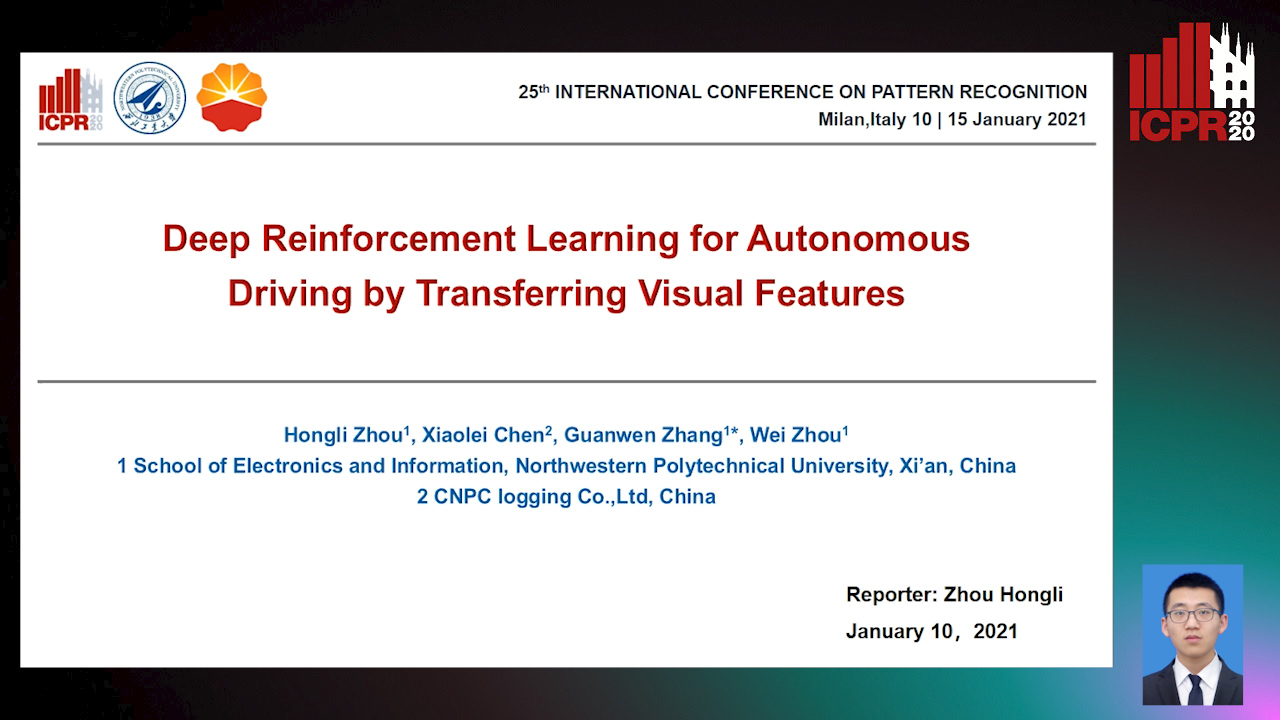
Auto-TLDR; Deep Reinforcement Learning for Autonomous Driving by Transferring Visual Features
Abstract Slides Poster Similar
Deep reinforcement learning (DRL) has achieved great success in processing vision-based driving tasks. However, the end-to-end training manner makes DRL agents suffer from overfitting training scenes. The agents easily fail to generalize to unseen environments. In this paper, we propose a deep reinforcement learning for autonomous driving by transferring visual features. We formulate the DRL training as a perception and control module and introduce adversarial training mechanism for autonomous driving. The perception module is able to extract invariant features between different domains through adversarial training. While the DRL agent can then be trained on the basis of low dimensional states. In this manner, the proposed approach enables trained agents to adapt to unseen environments by learning robust features invariant across various scenes. We evaluate the proposed approach by transferring visual features between different simulators. The experimental results demonstrate the driving policy trained in the source domain can be directly applied in the target domain, and achieve great efficient and effective performance for autonomous driving.