Pramuditha Perera
Paper download is intended for registered attendees only, and is
subjected to the IEEE Copyright Policy. Any other use is strongly forbidden.
Papers from this author
A Joint Representation Learning and Feature Modeling Approach for One-Class Recognition
Pramuditha Perera, Vishal Patel
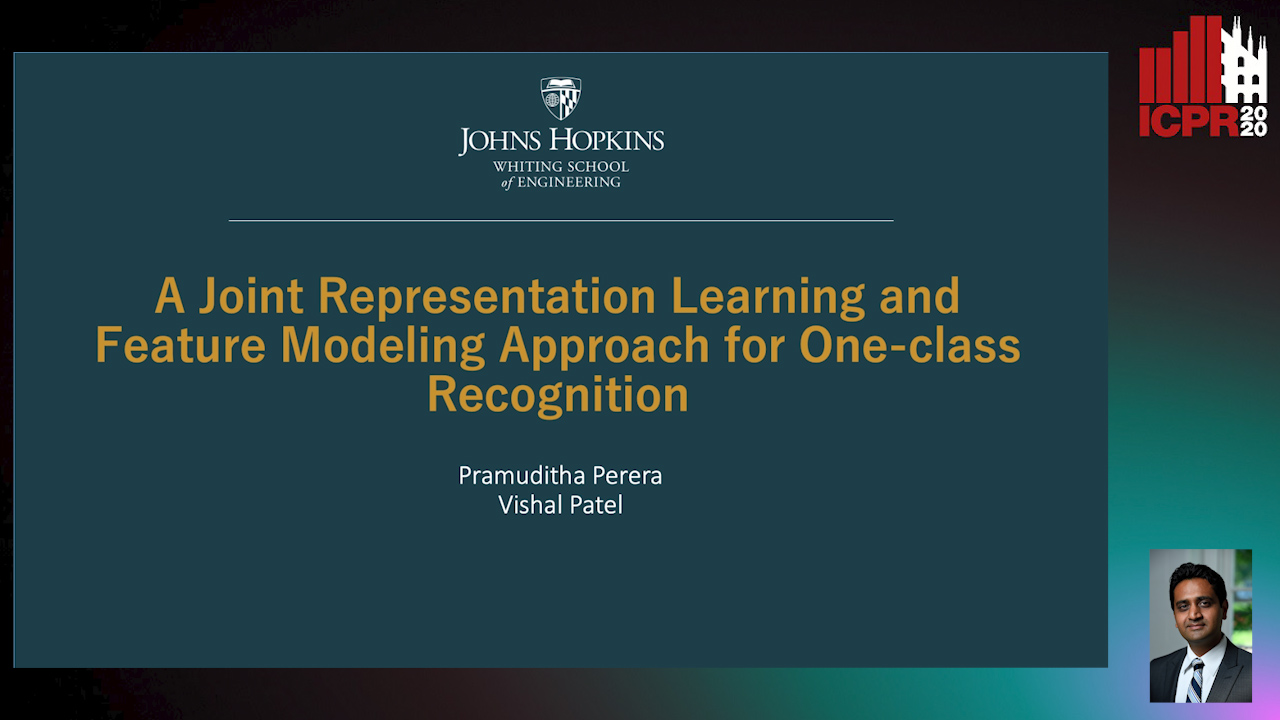
Auto-TLDR; Combining Generative Features and One-Class Classification for Effective One-class Recognition
Abstract Slides Poster Similar
One-class recognition is traditionally approached either as a representation learning problem or a feature modelling problem. In this work, we argue that both of these approaches have their own limitations; and a more effective solution can be obtained by combining the two. The proposed approach is based on the combination of a generative framework and a one-class classification method. First, we learn generative features using the one-class data with a generative framework. We augment the learned features with the corresponding reconstruction errors to obtain augmented features. Then, we qualitatively identify a suitable feature distribution that reduces the redundancy in the chosen classifier space. Finally, we force the augmented features to take the form of this distribution using an adversarial framework. We test the effectiveness of the proposed method on three one-class classification tasks and obtain state-of-the-art results.