Oyebade Kayode Oyedotun
Paper download is intended for registered attendees only, and is
subjected to the IEEE Copyright Policy. Any other use is strongly forbidden.
Papers from this author
Revisiting the Training of Very Deep Neural Networks without Skip Connections
Oyebade Kayode Oyedotun, Abd El Rahman Shabayek, Djamila Aouada, Bjorn Ottersten
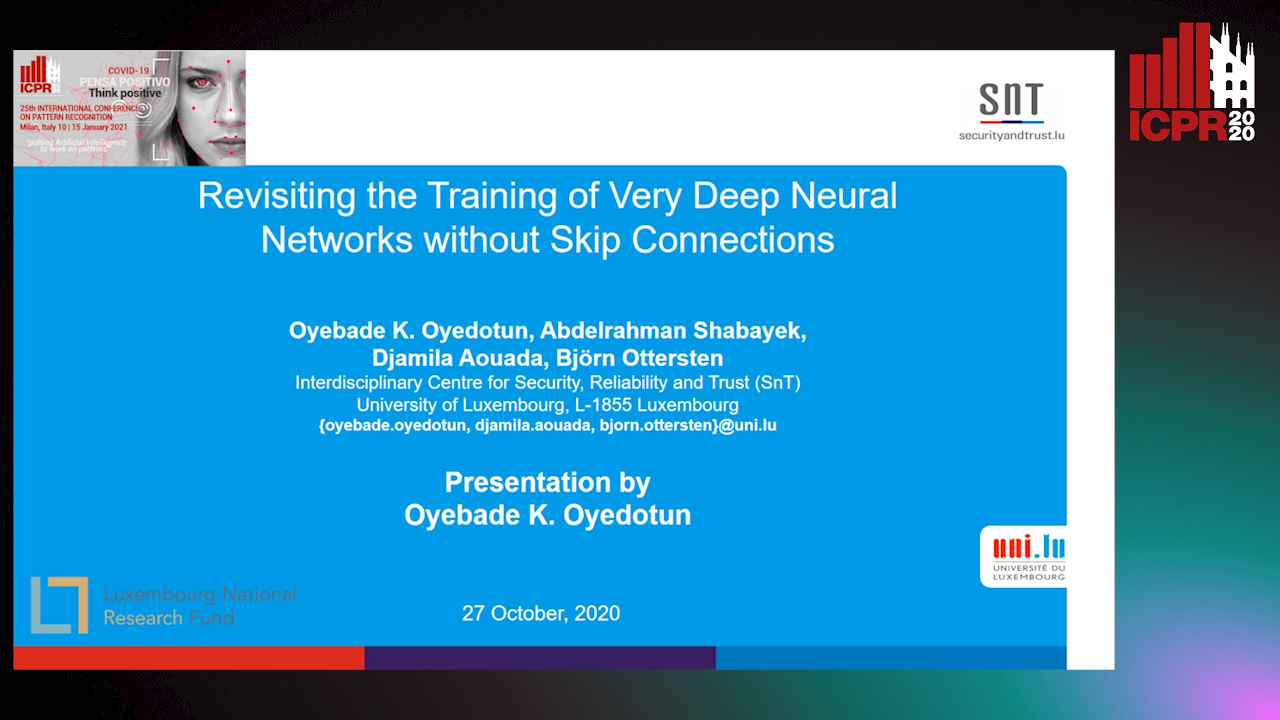
Auto-TLDR; Optimization of Very Deep PlainNets without shortcut connections with 'vanishing and exploding units' activations'
Abstract Slides Poster Similar
Deep neural networks (DNNs) with many layers of feature representations yield state-of-the-art results on several difficult learning tasks. However, optimizing very deep DNNs without shortcut connections known as PlainNets, is a notoriously hard problem. Considering the growing interest in this area, this paper investigates holistically two scenarios that plague the training of very deep PlainNets: (1) the relatively popular challenge of 'vanishing and exploding units' activations', and (2) the less investigated 'singularity' problem, which is studied in details in this paper. In contrast to earlier works that study only the saturation and explosion of units' activations in isolation, this paper harmonizes the inconspicuous coexistence of the aforementioned problems for very deep PlainNets. Particularly, we argue that the aforementioned problems would have to be tackled simultaneously for the successful training of very deep PlainNets. Finally, different techniques that can be employed for tackling the optimization problem are discussed, and a specific combination of simple techniques that allows the successful training of PlainNets having up to 100 layers is demonstrated.